12 Data Wrangling: A case Study
Case Study: What uniform pieces are we waiting for?
A volunteer with a children’s soccer team was trying to keep track of which child ordered which piece of equipment, what the size was, and whether the item was received. The team uses Excel, and the following Excel file was received:
[NOTE: INSERT EXCEL SHEET]
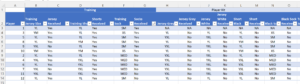
Note that this is a well organized table, but using it, it’s difficult to see how many pairs of Youth Median (YM) black shorts are still outstanding. This is a job for data wrangling – organizing the data in a way that makes our work clearer.
Tables should be long – not wide
This table is short and wide. There is a row for each child (identified by their jersey number) and columns for each item, item size, and status (received or not). The solution in this case was to wrangle this data in R, creating a long but narrow table.
Open the libraries we will need:
In the boxes you will find all of the R code needed for this project. Note that that this supposes that we have the `soccer.xlsx` file in our working directory
library(dplyr) ## dplyr is for working with data frames
library(openxlsx) ## lets us liaise with Excel library(stringr) ## Extract words to make it faster! ## read in the data
df <- read.xlsx("soccer.xlsx", sheet = "Detailorders", startRow = 2)
## Inspecting the data
Exploratory Data Analysis (EDA) is a fancy word for what we naturally do: look at the data and explore. Here’s what happens when we look around. First we look at what the structure of the data is:
df %>% str()
## 'data.frame': 12 obs. of 28 variables:
## $ Player : chr "1" "3" "5" "8" ...
## $ Training.Jersey.Size : chr "YL" "YM" "YL" "YL" ...
## $ Jersey.Received : chr "Yes" "Yes" "Yes" "Yes" ...
## $ Training.Short : chr "YL" "YL" "YL" "YM" ...
## $ Shorts.Received : chr "Yes" "Yes" "Yes" "Yes" ...
## $ Training.Sock : chr "SM" "XS" "SM" "SM" ...
## $ Socks.Received : chr "Yes" "Yes" "Yes" "Yes" ...
## $ Jersey.Grey : chr NA "YL" "YXL" "YL" ...
## $ Jersey.Grey.Received : chr NA "No" "No" "No" ...
## $ Jersey.White : chr NA "YL" "YXL" "YL" ...
## $ Jersey.White.Received: chr NA "No" "No" "No" ...
## $ Short.Black : chr NA "YL" "YL" "YM" ...
## $ Short.Received : chr NA "No" "No" "No" ...
## $ Black.Sock : chr NA "XS" "SM" "SM" ...
## $ Black.Sock.Received : chr NA "No" "No" "No" ...
## $ Track.Jacket : chr "YL" "YL" "YXL" "YL" ...
## $ Track.Jacket.Received: chr "No" "No" "No" "No" ...
## $ Track.Pant : chr "YL" "YL" "YL" "YL" ...
## $ Track.Pant.Received : chr "No" "No" "No" "No" ...
## $ Cinch.Pack : chr "Ordered " "Ordered " "Ordered " "Ordered " ...
## $ Cinch.Pack.Received : chr "No" "No" "No" "No" ...
## $ GK.Jersey : chr "YL" NA NA NA ...
## $ GK.Jersey.Received : chr "No" "No" "No" "No" ...
## $ GK.Short : chr "YL" NA NA NA ...
## $ GK.Short.Received : chr "No" "No" "No" "No" ...
## $ GK.Socks : chr "SM" NA NA NA ...
## $ GK.Sock.Received : chr "No" "No" "No" "No" ...
## $ Comments : chr NA NA NA " " ...
We will also take a minute to look at the data frame that we have:
df
Reshape the data:
We want to create a table that is narrow (not too many columns) and long (many rows). The way to do this is to create a row for each piece of equipment ordered. In the end we should have data that looks like this:
Player | Item | Size | Received |
---|---|---|---|
1 | Jersey | YL | Yes |
7 | Socks | Med | No |
There are many ways to do this, but here is one method:
## make a string of names
col_names <- colnames(df)
## cut the entry level stuff:
interm_list <- col_names[6:31]
interm_list
## [1] "Training.Jersey.Size" "Jersey.Received"
## [3] "Training.Short" "Shorts.Received"
## [5] "Training.Sock" "Socks.Received"
## [7] "Jersey.Grey" "Jersey.Grey.Received"
## [9] "Jersey.White" "Jersey.White.Received"
## [11] "Short.Black" "Short.Received"
## [13] "Black.Sock" "Black.Sock.Received"
## [15] "Track.Jacket" "Track.Jacket.Received"
## [17] "Track.Pant" "Track.Pant.Received"
## [19] "Cinch.Pack" "Cinch.Pack.Received"
## [21] "GK.Jersey" "GK.Jersey.Received"
## [23] "GK.Short" "GK.Short.Received"
## [25] "GK.Socks" "GK.Sock.Received"
## What are the sizes?
size_list <- c()
rec_list <- c()
for (i in 1:13){
size_list <- c(size_list,interm_list[2*i-1])
rec_list <- c(rec_list,interm_list[2*i])
}
item_list <- str_remove(rec_list,".Received")
## Make a blank dataframe
Soccer_df <- data.frame("Player"=numeric(), "Item"= character(), "Size"=character(), "Recieved"=character())
for (i in 1:13){
newdf <- df %>%
select(Player, size_list[i], rec_list[i])
names(newdf) <- c("Player", "Size", "Received")
newdf$Item <- item_list[i] newdf <- newdf %>%
select(Player, Item, Size, Received)
Soccer_df <- rbind(Soccer_df, newdf)
}
Inspect the first 5 rows:
Soccer_df %>% head()
## Player Item Size Received
## 1 1 Jersey YL Yes
## 2 3 Jersey YM Yes
## 3 5 Jersey YL Yes
## 4 8 Jersey YL Yes
## 5 9 Jersey YXL No
## 6 10 Jersey YM Yes
Now we will look at grouping the data.
I filtered out anything where the size was “NA”, as we assume that means that there is no item ordered.
summary <- Soccer_df %>%
filter(!is.na(Size))
%>%
group_by(Item, Size, Received)
%>%
summarize(Count = n())
to_save <- Soccer_df
%>%
filter(!is.na(Size))
%>%
group_by(Item, Size, Received)
%>%
summarize(Count = n())
%>%
filter(Received == "No")
to_save
## # A tibble: 23 x 4
## # Groups: Item, Size [23]
## Item Size Received Count
## <chr> <chr> <chr> <int>
## 1 Black.Sock MED No 5
## 2 Black.Sock SM No 4
## 3 Black.Sock XS No 2
## 4 Cinch.Pack "Ordered " No 12
## 5 GK.Jersey YL No 1
## 6 GK.Short YL No 1
## 7 GK.Sock SM No 1
## 8 Jersey YXL No 3
## 9 Jersey.Grey YL No 4
## 10 Jersey.Grey YXL No 7
## # ... with 13 more rows
Save
Finally, we cansave that file as a new Excel sheet – or you could mkae it an old Excel Sheet, whatever!
Learning Objectives
file_name <- paste0("Soccer_",Sys.Date(),".xlsx")
file_name
## [1] "Soccer_2022-04-08.xlsx"
wb <- createWorkbook("Soccer_Gear")
addWorksheet(wb, sheetName = "original")
writeData(wb, sheet = "original", x = df)
addWorksheet(wb, sheetName = "Not_Received")
writeData(wb, sheet = "Not_Received", x = to_save)
addWorksheet(wb, sheetName = "Summary")
writeData(wb, sheet = "Summary", x = summary)
saveWorkbook(wb, file_name, overwrite = TRUE)
## Player Training.Jersey.Size Jersey.Received Training.Short Shorts.Received
## 1 1 YL Yes YL Yes
## 2 3 YM Yes YL Yes
## 3 5 YL Yes YL Yes
## 4 8 YL Yes YM Yes
## 5 9 YXL No YL Yes
## 6 10 YM Yes YM Yes
## 7 2 YL Yes YL Yes
## 8 4 YXL Yes YXL Yes
## 9 6 YL Yes YXL Yes
## 10 7 YXL No YXL Yes
## 11 11 YXL No YXL Yes
## 12 12 YL Yes YL Yes
## Training.Sock Socks.Received Jersey.Grey Jersey.Grey.Received Jersey.White
## 1 SM Yes <NA> <NA> <NA>
## 2 XS Yes YL No YL
## 3 SM Yes YXL No YXL
## 4 SM Yes YL No YL
## 5 SM Yes YXL No YXL
## 6 XS Yes YL No YL
## 7 MED No YXL No YXL
## 8 MED No YXL No YXL
## 9 MED No YXL No YXL
## 10 MED No YXL No YXL
## 11 MED No YXL No YXL
## 12 SM No YL No YL
## Jersey.White.Received Short.Black Short.Received Black.Sock
## 1 <NA> <NA> <NA> <NA>
## 2 No YL No XS
## 3 No YL No SM
## 4 No YM No SM
## 5 No YL No SM
## 6 No YM No XS
## 7 No YL No MED
## 8 No YXL No MED
## 9 No YXL No MED
## 10 No YXL No MED
## 11 No YXL No MED
## 12 No YL No SM
## Black.Sock.Received Track.Jacket Track.Jacket.Received Track.Pant
## 1 <NA> YL No YL
## 2 No YL No YL
## 3 No YXL No YL
## 4 No YL No YL
## 5 No YL No YL
## 6 No YL No YM
## 7 No YL No YL
## 8 No YL No YL
## 9 No YXL No YL
## 10 No YXL No YL
## 11 No YXL No YXL
## 12 No YM No YL
## Track.Pant.Received Cinch.Pack Cinch.Pack.Received GK.Jersey
## 1 No Ordered No YL
## 2 No Ordered No <NA>
## 3 No Ordered No <NA>
## 4 No Ordered No <NA>
## 5 No Ordered No <NA>
## 6 No Ordered No <NA>
## 7 No Ordered No <NA>
## 8 No Ordered No <NA>
## 9 No Ordered No <NA>
## 10 No Ordered No <NA>
## 11 No Ordered No <NA>
## 12 No Ordered No <NA>
## GK.Jersey.Received GK.Short GK.Short.Received GK.Socks GK.Sock.Received
## 1 No YL No SM No
## 2 No <NA> No <NA> No
## 3 No <NA> No <NA> No
## 4 No <NA> No <NA> No
## 5 No <NA> No <NA> No
## 6 No <NA> No <NA> No
## 7 No <NA> No <NA> No
## 8 No <NA> No <NA> No
## 9 No <NA> No <NA> No
## 10 No <NA> No <NA> No
## 11 No <NA> No <NA> No
## 12 No <NA> No <NA> No
## Comments
## 1 <NA>
## 2 <NA>
## 3 <NA>
## 4
## 5 Never received jersey
## 6 <NA>
## 7 Need size 2 socks
## 8 Need size 2 socks
## 9 Need size 2 socks
## 10 Never received jersey , Need Siz 2 sock
## 11 Never received jersey, need size 2 socks
## 12 Need size 2 socks