10 Quantitative sampling
Chapter Outline
- The sampling process (25 minute read)
- Sampling approaches for quantitative research (15 minute read)
- Sample quality (24 minute read)
Content warning: examples contain references to addiction to technology, domestic violence and batterer intervention, cancer, illegal drug use, LGBTQ+ discrimination, binge drinking, intimate partner violence among college students, child abuse, neocolonialism and Western hegemony.
10.1 The sampling process
Learning Objectives
Learners will be able to…
- Decide where to get your data and who you might need to talk to
- Evaluate whether it is feasible for you to collect first-hand data from your target population
- Describe the process of sampling
- Apply population, sampling frame, and other sampling terminology to sampling people in your project’s target population
One of the things that surprised me most as a research methods professor is how much my students struggle with understanding sampling. It is surprising because people engage in sampling all the time. How do you learn whether you like a particular food, like BBQ ribs? You sample them from different restaurants! Obviously, social scientists put a bit more effort and thought into the process than that, but the underlying logic is the same. By sampling a small group of BBQ ribs from different restaurants and liking most of them, you can conclude that when you encounter BBQ ribs again, you will probably like them. You don’t need to eat all of the BBQ ribs in the world to come to that conclusion, just a small sample.[1] Part of the difficulty my students face is learning sampling terminology, which is the focus of this section.
Who is your study about and who should you talk to?
At this point in the research process, you know what your research question is. Our goal in this chapter is to help you understand how to find the people (or documents) you need to study in order to find the answer to your research question. It may be helpful at this point to distinguish between two concepts. Your unit of analysis is the entity that you wish to be able to say something about at the end of your study (probably what you’d consider to be the main focus of your study). Your unit of observation is the entity (or entities) that you actually observe, measure, or collect in the course of trying to learn something about your unit of analysis.
It is often the case that your unit of analysis and unit of observation are the same. For example, we may want to say something about education students (unit of analysis), so we ask education students at our university to complete a survey for our study (unit of observation). In this case, we are observing individuals, i.e. students, so we can make conclusions about individuals.
On the other hand, our unit of analysis and observation can differ. We could sample students to draw conclusions about organizations or universities. Perhaps we are comparing students at universities and colleges. Even though our sample was made up of individual students from various post secondary institutions (our unit of observation), our unit of analysis was the university or college as an organization. Conclusions we made from individual-level data were used to understand larger organizations.
Similarly, we could adjust our sampling approach to target specific student cohorts. Perhaps we wanted to understand the experiences of Indigenous education students at BC universities. We could choose either an individual unit of observation by selecting Indigenous students, or a group unit of observation by studying members of an organization, like the Aboriginal Education Association within the BCTF.
Sometimes the units of analysis and observation differ due to pragmatic reasons. If we wanted to study whether being an education student impacted family relationships, we may choose to study family members of students in education programs who could give us information about how they behaved in the home. In this case, we would be observing family members to draw conclusions about individual students.
In sum, there are many potential units of analysis that a researcher might examine, but some of the most common include individuals, groups, and organizations. Table 10.1 details examples identifying the units of observation and analysis in a hypothetical study of student addiction to electronic gadgets.
Research question | Unit of analysis | Data collection | Unit of observation | Statement of findings |
Which students are most likely to be addicted to their electronic gadgets? | Individuals | Survey of students on campus | Individuals | New Media majors, men, and students with high socioeconomic status are all more likely than other students to become addicted to their electronic gadgets. |
Do certain types of social clubs have more gadget-addicted members than other sorts of clubs? | Groups | Survey of students on campus | Individuals | Clubs with a scholarly focus, such as social work club and the math club, have more gadget-addicted members than clubs with a social focus, such as the 100-bottles-of- beer-on-the-wall club and the knitting club. |
How do different colleges address the problem of electronic gadget addiction? | Organizations | Content analysis of policies | Documents | Campuses without strong computer science programs are more likely than those with such programs to expel students who have been found to have addictions to their electronic gadgets. |
Note: Please remember that the findings described here are hypothetical. There is no reason to think that any of the hypothetical findings described here would actually bear out if empirically tested. |
First-hand vs. second-hand knowledge
Your unit of analysis will be determined by your research question. Specifically, it should relate to your target population. Your unit of observation, on the other hand, is determined largely by the method of data collection you use to answer that research question. Let’s consider a common issue in education research: understanding the effectiveness of different classroom interventions. Who has first-hand knowledge and who has second-hand knowledge? Well, practitioners would have first-hand knowledge about implementing the intervention. For example, they might discuss with you the unique language they use help students understand the intervention. Students, on the other hand, have first-hand knowledge about the impact of those interventions on their learning. If you want to know if an intervention is effective, you need to ask people who have received it!
Unfortunately, student projects run into pragmatic limitations with sampling from student groups. Younger students are considered a vulnerable population at greater risk of harm. Asking a person who was recently experiencing bullying in school about that experience may cause trauma as the relive painful experiences. Asking one’s own students to participate in the study creates a dual relationship with the student, as both teacher and researcher, and dual relationships have conflicting responsibilities and boundaries.
Obviously, studies are done with students all the time. But for your projects in the classroom, it is often required to get second-hand information from a population that is less vulnerable. Students may instead choose to study other educators and how they perceive the effectiveness of different interventions. While teachers can provide an informed perspective, they have less knowledge about personally receiving the intervention. In general, researchers prefer to sample the people who have first-hand knowledge about their topic, though feasibility often forces them to analyze second-hand information instead.
Population: Who do you want to study?
In social scientific research, a population is the cluster of people you are most interested in. It is often the “who” that you want to be able to say something about at the end of your study. While populations in research may be rather large, such as “the American people,” they are typically more specific than that. For example, a large study for which the population of interest is the American people will likely specify which American people, such as adults over the age of 18 or citizens or legal permanent residents. Based on your work in Chapter 2, you should have a target population identified in your working question. That might be something like “people with developmental disabilities” or “students in a teacher education program.”
It is almost impossible for a researcher to gather data from their entire population of interest. This might sound surprising or disappointing until you think about the kinds of research questions that we typically ask. For example, let’s say we wish to answer the following question: “How does gender impact attendance in elementary school?” Would you expect to be able to collect data from all students in all elementary schools across all nations from all historical time periods? Unless you plan to make answering this research question your entire life’s work (and then some), I’m guessing your answer is a resounding no. So, what to do? Does not having the time or resources to gather data from every single person of interest mean having to give up your research interest?
Exercises
Let’s think about who could possibly be in your study.
- What is your population, the people you want to make conclusions about?
- Do your unit of analysis and unit of observation differ or are they the same?
- Can you ethically and practically get first-hand information from the people most knowledgeable about the topic, or will you rely on second-hand information from less vulnerable populations?
Setting: Where will you go to get your data?
While you can’t gather data from everyone, you can find some people from your target population to study. The first rule of sampling is: go where your participants are. You will need to figure out where you will go to get your data. For many student researchers, it is their school, their peers, their family and friends, or whoever comes across students’ social media posts or emails asking people to participate in their study.
Each setting (school, social media) limits your reach to only a small segment of your target population who has the opportunity to be a part of your study. This intermediate point between the overall population and the sample of people who actually participate in the researcher’s study is called a sampling frame. A sampling frame is a list of people from which you will draw your sample.
But where do you find a sampling frame? Answering this question is the first step in conducting human subjects research. Education researchers must think about locations or groups in which your target population gathers or interacts. For example, a study on quality of instruction in middle schools may choose a middle school because it’s easy to access. The sampling frame could be all of the teachers in the school. You would select your participants for your study from the list of teachers in the school. Note that this is a real list. That is, an administrator at the school would give you a list with every teacher’s name and contact information from which you would select your participants. If you decided to include more schools in your study, then your sampling frame could be all the teachers at all the schools who agreed to participate in your study.
Let’s consider some more examples. Unlike current middle school teachers in a specific school, you might select teachers engaging in particular professional development. For researchers to reach these participants (say, on a study of teacher wellness), they may consider partnering with a school district or professional body (like the BCTF) to find professional development day offerings focused on teacher wellness. Without a set list of people, your sampling frame would simply be the people who showed up to the PD offering on the day you were there. Similarly, if you posted an advertisement in an online peer-support group for teachers experiencing stress, your sampling frame is the people in that group.
More challenging still is recruiting people who are homeless, those with very low income, or those who belong to stigmatized groups. For example, a research study by Johnson and Johnson (2014)[2] attempted to learn usage patterns of “bath salts,” or synthetic stimulants that are marketed as “legal highs.” Users of “bath salts” don’t often gather for meetings, and reaching out to individual treatment centers is unlikely to produce enough participants for a study, as the use of bath salts is rare. To reach participants, these researchers ingeniously used online discussion boards in which users of these drugs communicate. Their sampling frame included everyone who participated in the online discussion boards during the time they collected data. Another example might include using a flyer to let people know about your study, in which case your sampling frame would be anyone who walks past your flyer wherever you hang it—usually in a strategic location where you know your population will be (for example, the teachers room in a school or at the registration table at the New Teachers’ Conference 2022).
In conclusion, sampling frames can be a real list of people like the list of faculty in a university department, which allows you to clearly identify who is in your study and what chance they have of being selected. However, not all sampling frames allow you to be so specific. It is also important to remember that accessing your sampling frame must be practical and ethical, as we discussed in Chapter 2 and Chapter 6. For studies that present risks to participants, approval from gatekeepers and the university’s institutional review board (IRB) is needed.
Criteria: What characteristics must your participants have/not have?
Your sampling frame is not just everyone in the setting you identified. For example, if you were studying education students who are first-generation university students, you might select your university as the setting, but not everyone in your program is a first-generation student. You need to be more specific about which characteristics or attributes individuals either must have or cannot have before they participate in the study. These are known as inclusion and exclusion criteria, respectively.
Inclusion criteria are the characteristics a person must possess in order to be included in your sample. If you were conducting a survey on LGBTQ+ discrimination in your school district, you might want to sample only employees who identify as LGBTQ+. In that case, your inclusion criteria for your sample would be that individuals have to identify as LGBTQ+.
Comparably, exclusion criteria are characteristics that disqualify a person from being included in your sample. In the previous example, you could think of cisgenderism and heterosexuality as your exclusion criteria because no person who identifies as heterosexual or cisgender would be included in your sample. Exclusion criteria are often the mirror image of inclusion criteria. However, there may be other criteria by which we want to exclude people from our sample. For example, we may exclude employees who were recently hired (since they don’t have experience working in the district yet).
Recruitment: How will you ask people to participate in your study?
Once you have a location and list of people from which to select, all that is left is to reach out to your participants. Recruitment refers to the process by which the researcher informs potential participants about the study and asks them to participate in the research project. Recruitment comes in many different forms. If you have ever received a phone call asking for you to participate in a survey, someone has attempted to recruit you for their study. Perhaps you’ve seen print advertisements on buses, in student centers, or in a newspaper. I’ve received many emails that were passed around my school asking for participants, usually for a graduate student project. As we learn more about specific types of sampling, make sure your recruitment strategy makes sense with your sampling approach. For example, if you put up a flyer in the student health office to recruit student athletes for your study, you may not be targeting your recruitment efforts to settings where your target population is likely to see your recruitment materials (unless you’re looking for student athletes receiving health services from the university).
Recruiting human participants
Sampling is the first time in which you will contact potential study participants. Before you start this process, it is important to make sure you have approval from your university’s institutional review board as well as any gatekeepers at the locations in which you plan to conduct your study (like principals or district superintendents). As we discussed in section 10.1, the first rule of sampling is to go where your participants are. If you are studying domestic violence, reach out to local shelters, advocates, or service agencies. Gatekeepers will be necessary to gain access to your participants. For example, a gatekeeper can forward your recruitment email across their employee email list. Review our discussion of gatekeepers in Chapter 2 before proceeding with contacting potential participants as part of recruitment.
Recruitment can take many forms. You may show up at a staff meeting in your school to ask for volunteers. You may send a school-wide email. Each step of this process should be vetted by the IRB (and your supervisor) as well as other stakeholders and gatekeepers. You will also need to set reasonable expectations for how many reminders you will send to the person before moving on. Generally, it is a good idea to give people a little while to respond, though reminders are often accompanied by an increase in participation. Pragmatically, it is a good idea for you to think through each step of the recruitment process and how much time it will take to complete it.
For example, I was interested in understanding the sources of information school trustees consulted when they wanted to get information for making decisions about their school district. To access trustees, it helped for me to bring on board a former trustee as a coauthor and research partner who had unique knowledge about the group and how best to contact them. She also reviewed the survey items to make sure they fit the BC context and would gather the type of information we wanted. She also help reach out to previous colleagues who could help us pilot test the survey. The sampling frame was all current trustees who we could find contact information for through public databases (412). This collaborative process took time and had to be completed before data collection could start. Once sampling commenced, I sent an e-mail message to each BC school trustee, followed up by a reminder message a few weeks later, and finally a survey closing message three days before we concluded the data gathering. The process took months to complete. As an added benefit, partnering with a former trustee meant that we were able to share our work with the BCSTA, which helped disseminate the research to their members in a more accessible format than our traditional journal publications.
Recruitment will also expose your participants to the informed consent information you prepared. For students going through the IRB, there are templates you will have to follow in order to get your study approved. In the aforementioned study, I used our IRB’s template to create a consent form but did not include a signature line. The IRB allowed me to collect my data without a signature, as there was little risk of harm from the study and participants had to actively click a link to access the survey. It was imperative to review consent information before completing the survey with participants. Only when the participant is totally clear on the purpose, risks and benefits, confidentiality protections, and other information detailed in Chapter 6, can you ethically move forward with including them in your sample.
Sampling available documents
As with sampling humans, sampling documents centers around the question: which documents are the most relevant to your research question, in that which will provide you first-hand knowledge. Common documents analyzed in student research projects include mission statements, web sites, popular media like film and music lyrics, and levitation and policies from government agencies. In some cases researchers might be accessing student files, employment records, or assessment data. In a case record review, researchers would create exclusion and inclusion criteria based on their research question. Once a suitable sampling frame of potential documents existed, the researcher would use probability or non-probability sampling to select which files are ultimately analyzed.
Sampling documents must also come with consent and buy-in from stakeholders and gatekeepers. Assuming you have approval to conduct your study and access to the documents you need, the process of recruitment is much easier than in studies sampling humans. There is no informed consent process with documents, though research with confidential health or education records must be done in accordance with privacy laws such as the BC Freedom of Information Protection or Privacy Act and relevant federal laws. Barring any technical or policy obstacles, the gathering of documents can be easier and less time consuming than sampling humans.
Sample: Who actually participates in your study?
Once you find a sampling frame from which you can recruit your participants and decide which characteristics you will include and exclude, you will recruit people using a specific sampling approach, which we will cover in Section 10.2. At the end, you’re left with the group of people you successfully recruited from your sampling frame to participate in your study, your sample. If you are a participant in a research project—answering survey questions, participating in interviews, etc.—you are part of the sample in that research project.
Visualizing sampling terms
Sampling terms can be a bit daunting at first. However, with some practice, they will become second nature. Let’s walk through an example from a research project of the original book’s author[3]. He collected data for a research project related to how much it costs to become a licensed clinical social worker (LCSW) in each state of the US. Becoming an LCSW is necessary to work in private clinical practice and is used by supervisors in human service organizations to sign off on clinical charts from less credentialed employees, and to provide clinical supervision.
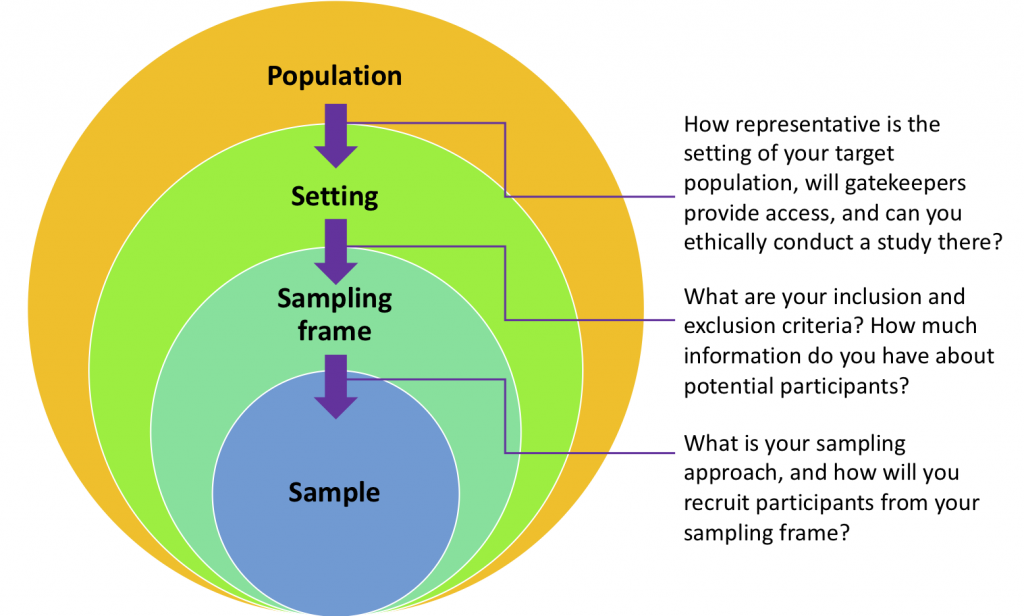
Using Figure 10.1 as a guide, his population is clearly clinical social workers, as these are the people about whom he wants to draw conclusions. The next step inward would be a sampling frame. Unfortunately, there is no list of every licensed clinical social worker in the United States. He could write to each state’s social work licensing board and ask for a list of names and addresses, perhaps even using a Freedom of Information Act request if they were unwilling to share the information. That option sounds time-consuming and has a low likelihood of success. Instead, he tried to figure out a convenient setting where social workers are likely to congregate. He considered setting up a booth at a National Association of Social Workers (NASW) conference and asking people to participate in his survey. Ultimately, this would prove too costly, and the people who gather at an NASW conference may not be representative of the general population of clinical social workers. He finally discovered the NASW membership email list, which is available to advertisers, including researchers advertising for research projects. While the NASW list does not contain every clinical social worker, it reaches over one hundred thousand social workers regularly through its monthly e-newsletter, a large proportion of social workers in practice, so the setting was likely to draw a representative sample. To gain access to this setting from gatekeepers, he had to provide paperwork showing his study had undergone IRB review and submit his measures for approval by the mailing list administrator.
Once he gained access from gatekeepers, his setting became the members of the NASW membership list. He decided to try and recruit at least 5,000 participants because he knew that people sometimes do not read or respond to email advertisements, and he figured maybe 20% would respond, which would give him around 1,000 responses. Figuring out his sample size was a challenge, because he had to balance the costs associated with using the NASW newsletter. As you can see on their pricing page, it would cost money to learn personal information about his potential participants, which he would need to check later in order to determine if his population was representative of the overall population of social workers. For example, he could see if his sample was comparable in race, age, gender, or state of residence to the broader population of social workers by comparing his sample with information about all social workers published by NASW. He presented his options to his external funder as:
- I could send an email advertisement to a lot of people (5,000), but I would know very little about them and they would get only one advertisement.
- I could send multiple advertisements to fewer people (1,000) reminding them to participate, but I would also know more about them by purchasing access to personal information.
- I could send multiple advertisements to fewer people (2,500), but not purchase access to personal information to minimize costs.
They decided to go with option #1. When he sent his email recruiting participants for the study, he specified that he only wanted to hear from social workers who were either currently receiving or recently received clinical supervision for licensure—his inclusion criteria. This was important because many of the people on the NASW membership list may not be licensed or license-seeking social workers. So, his sampling frame was the email addresses on the NASW mailing list who fit the inclusion criteria for the study, which he figured would be at least a few thousand people. Unfortunately, only 150 licensed or license-seeking clinical social workers responded to his recruitment email and completed the survey. You will learn in Section 10.3 why this did not make for a very good sample. That said, you’ll note that he completed the study. Even if your study doesn’t go as planned there are always options for moving forward.
From this example, you can see that sampling is a process. The process flows sequentially from figuring out your target population, to thinking about where to find people from your target population, to figuring out how much information you know about potential participants, and finally to selecting people to recruit from that list to be a part of your sample. Through the sampling process, you must consider where people in your target population are likely to be and how best to get their attention for your study. Sampling can be an easy process, like calling every 100th name from the phone book, or challenging, like standing every day for a few weeks in an area in which people who are homeless gather for shelter. In either case, your goal is to recruit enough people who will participate in your study so you can learn about your population.
What about sampling non-humans?
Many student projects do not involve recruiting and sampling human subjects. Instead, many research projects will sample objects like charts, movies, or books. The same terms apply, but the process is a bit easier because there are no humans involved. If a research project involves analyzing student files, it is unlikely you will look at every student file that your school (or district) has. You will need to figure out which files are important to your research question. Perhaps you want to sample students who have a diagnosis of ADHD. You would have to create a list of all students at your school (setting) who have ADHD (your inclusion criteria) then use your sampling approach (which we will discuss in the next section) to select which files you will actually analyze for your study (your sample). Recruitment is a lot easier because, well, there’s no one to convince but your gatekeepers, the managers of your school and/pr district. However, researchers who publish document reviews must still obtain IRB permission before doing so.
Key Takeaways
- The first rule of sampling is to go where your participants are. Think about virtual or in-person settings in which your target population gathers. Remember that you may have to engage gatekeepers and stakeholders in accessing many settings, and that you will need to assess the pragmatic challenges and ethical risks and benefits of your study.
- Consider whether you can sample documents like agency files to answer your research question. Documents are much easier to “recruit” than people!
- Researchers must consider which characteristics are necessary for people to have (inclusion criteria) or not have (exclusion criteria), as well as how to recruit participants into the sample.
- Researchers can sample individuals, groups, or organizations.
- Sometimes the unit of analysis and the unit of observation in the study differ. In student projects, this is often true as target populations may be too vulnerable to expose to research whose potential harms may outweigh the benefits.
- One’s recruitment method has to match one’s sampling approach, as will be explained in the next chapter.
Exercises
Once you have identified who may be a part of your study, the next step is to think about where those people gather. Are there in-person locations in your community or on the internet that are easily accessible. List at least one potential setting for your project. Describe for each potential setting:
- Based on what you know right now, how representative of your population are potential participants in the setting?
- How much information can you reasonably know about potential participants before you recruit them?
- Are there gatekeepers and what kinds of concerns might they have?
- Are there any stakeholders that may be beneficial to bring on board as part of your research team for the project?
- What interests might stakeholders and gatekeepers bring to the project and would they align with your vision for the project?
- What ethical issues might you encounter if you sampled people in this setting.
Even though you may not be 100% sure about your setting yet, let’s think about the next steps.
10.2 Sampling approaches for quantitative research
Learning Objectives
Learners will be able to…
- Determine whether you will use probability or non-probability sampling, given the strengths and limitations of each specific sampling approach
- Distinguish between approaches to probability sampling and detail the reasons to use each approach
Sampling in quantitative research projects is done because it is not feasible to study the whole population, and researchers hope to take what we learn about a small group of people (your sample) and apply it to a larger population. There are many ways to approach this process, and they can be grouped into two categories—probability sampling and non-probability sampling. Sampling approaches are inextricably linked with recruitment, and researchers should ensure that their proposal’s recruitment strategy matches the sampling approach.
Probability sampling approaches use a random process, usually a computer program, to select participants from the sampling frame so that everyone has an equal chance of being included. It’s important to note that random means the researcher used a process that is truly random. In a project sampling college students, standing outside of the building in which your department is housed and surveying everyone who walks past is not random. Because of the location, you are likely to recruit a disproportionately large number of education students and fewer from other disciplines. Depending on the time of day, you may recruit more traditional undergraduate students, who take classes during the day, or more graduate students, who take classes in the evenings.
In this example, you are actually using non-probability sampling. Another way to say this is that you are using the most common sampling approach for student projects, availability sampling. Also called convenience sampling, this approach simply recruits people who are convenient or easily available to the researcher. If you have ever been asked by a friend to participate in their research study for their class or seen an advertisement for a study on a bulletin board or social media, you were being recruited using an availability sampling approach.
There are a number of benefits to the availability sampling approach. First and foremost, it is less costly and time-consuming for the researcher. As long as the person you are attempting to recruit has knowledge of the topic you are studying, the information you get from the sample you recruit will be relevant to your topic (although your sample may not necessarily be representative of a larger population). Availability samples can also be helpful when random sampling isn’t practical. If you are planning to survey students in an LGBTQ+ support group on campus but attendance varies from meeting to meeting, you may show up at a meeting and ask anyone present to participate in your study. A support group with varied membership makes it impossible to have a real list—or sampling frame—from which to randomly selected individuals. Availability sampling would help you reach that population.
Availability sampling is appropriate for student and smaller-scale projects, but it comes with significant limitations. The purpose of sampling in quantitative research is to generalize from a small sample to a larger population. Because availability sampling does not use a random process to select participants, the researcher cannot be sure their sample is representative of the population they hope to generalize to. Instead, the recruitment processes may have been structured by other factors that may bias the sample to be different in some way than the overall population.
So, for instance, if we asked education students about their level of satisfaction with the services at the library, and we sampled in the evenings, we would most likely get a biased perspective of the issue. Students taking only night classes are much more likely to commute to school, spend less time on campus, and use fewer campus services. Our results would not represent what all education students feel about the topic. We might get the impression that no education student had ever visited the library, when that is not actually true at all. Sampling bias will be discussed in detail in Section 10.3.
Approaches to probability sampling
What might be a better strategy is getting a list of all email addresses of education students and randomly selecting email addresses of students to whom you can send your survey. This would be an example of simple random sampling. It’s important to note that you need a real list of people in your sampling frame from which to select your email addresses. For projects where the people who could potentially participate are not known by the researcher, probability sampling is not possible. It is likely that administrators at your school’s registrar would be reluctant to share the list of students’ names and email addresses. Always remember to consider the feasibility and ethical implications of the sampling approach you choose.
Usually, simple random sampling is accomplished by assigning each person, or element, in your sampling frame a number and selecting your participants using a random number generator (Excel can be set up to do this). You would follow an identical process if you were sampling records or documents as your elements, rather than people. True randomness is difficult to achieve, and it takes complex computational calculations to do so. Although you think you can select things at random, human-generated randomness is actually quite predictable, as it falls into patterns called heuristics. To truly randomly select elements, researchers must rely on computer-generated help. Many free websites have good pseudo-random number generators. A good example is the website Random.org, which contains a random number generator that can also randomize lists of participants. Sometimes, researchers use a table of numbers that have been generated randomly. There are several possible sources for obtaining a random number table. Some statistics and research methods textbooks provide such tables in an appendix.
Though simple, this approach to sampling can be tedious since the researcher must assign a number to each person in a sampling frame. Systematic sampling techniques are somewhat less tedious but offer the benefits of a random sample. As with simple random samples, you must possess a list of everyone in your sampling frame. Once you’ve done that, to draw a systematic sample you’d simply select every kth element on your list. But what is k, and where on the list of population elements does one begin the selection process?
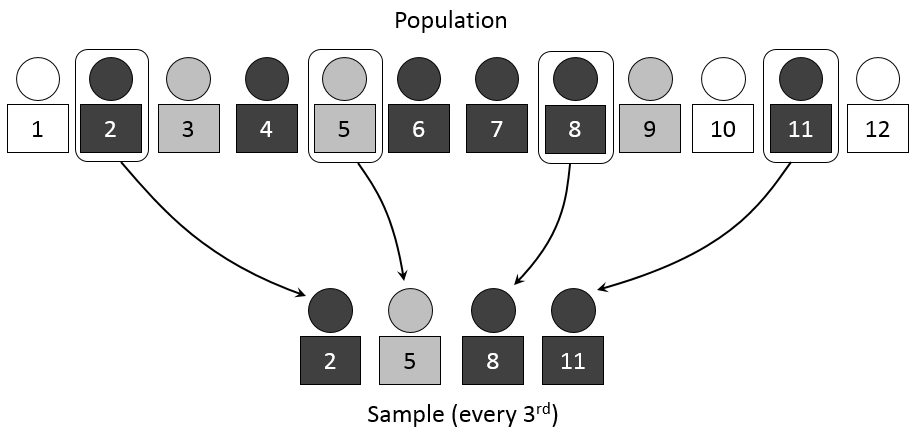
k is your selection interval or the distance between the elements you select for inclusion in your study. To begin the selection process, you’ll need to figure out how many elements you wish to include in your sample. Let’s say you want to survey 25 teachers and there are 75 on your school campus. In this case, your selection interval, or k, is 3. To get your selection interval, simply divide the total number of population elements by your desired sample size. Systematic sampling starts by randomly selecting a number between 1 and k to start from, and then recruiting every kth person. In our example, we may start at number 2 and then select the 5th, 8th, 11th (and so forth) person on our list of email addresses. In Figure 10.2, you can see the researcher starts at number 2 and then selects every third person for inclusion in the sample.
There is one clear instance in which systematic sampling should not be employed. If your sampling frame has any pattern to it, you could inadvertently introduce bias into your sample by using a systemic sampling strategy. (Bias will be discussed in more depth in section 10.3.) This is sometimes referred to as the problem of periodicity. Periodicity refers to the tendency for a pattern to occur at regular intervals.
To stray a bit from our example, imagine we were sampling student absences over the school year based on the date they missed school and recording the reason for their absence. We may expect more excused absences on Mondays and Fridays than we would during the rest of the week. The periodicity of absences may bias our sample towards either overrepresenting or underrepresenting this issue, depending on our sampling interval and whether we collected data on a particular weekday (or set of weekdays).
Advanced probability sampling techniques
Returning again to our idea of sampling student email addresses, one of the challenges in our study will be the different types of students. If we are interested in all education students, it may be helpful to divide our sampling frame, or list of students, into three lists—one for traditional, full-time undergraduate students, another for part-time undergraduate students, and one more for full-time graduate students—and then randomly select from these lists. This is particularly important if we wanted to make sure our sample had the same proportion of each type of student compared with the general population.
This approach is called stratified random sampling. In stratified random sampling, a researcher will divide the study population into relevant subgroups or strata and then draw a sample from each subgroup, or stratum. Strata is the plural of stratum, so it refers to all of the groups while stratum refers to each group. This can be used to make sure your sample has the same proportion of people from each stratum. If, for example, our sample had many more graduate students than undergraduate students, we may draw incorrect conclusions that do not represent what all education students experience.
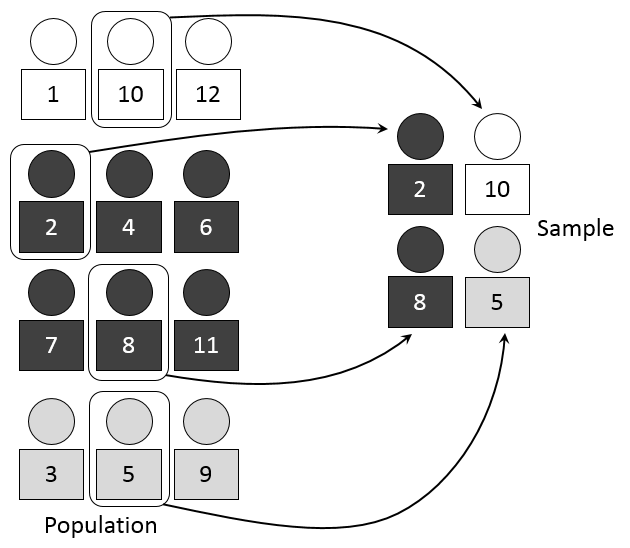
Generally, the goal of stratified random sampling is to recruit a sample that makes sure all elements of interest of the population are included sufficiently that conclusions can be drawn about them. Usually, the purpose is to create a sample that is identical to the overall population along whatever strata you’ve identified. In our sample, it would be graduate and undergraduate students. Stratified random sampling is also useful when a subgroup of interest makes up a relatively small proportion of the overall sample. For example, if your education program may contain relatively few Indigenous students but you wanted to make sure you recruited enough Indigenous students to conduct statistical analysis. You could use self-declared ethnicity to divide people into subgroups or strata and then disproportionately sample from the Indigenous students to make sure enough of them were in your sample to draw meaningful conclusions. Many statistical tests have a minimum number for accurate analysis.
Up to this point in our discussion of probability samples, we’ve assumed that researchers will be able to access a list of population elements in order to create a sampling frame. This, as you might imagine, is not always the case. Let’s say, for example, that you wish to conduct a study of library usage across elementary-age students at each school in your your province. Just imagine trying to create a list of every single student in the province. Even if you could find a way to generate such a list, attempting to do so might not be the most practical use of your time or resources. When this is the case, researchers turn to cluster sampling. Cluster sampling occurs when a researcher begins by sampling groups (or clusters) of population elements and then selects elements from within those groups.
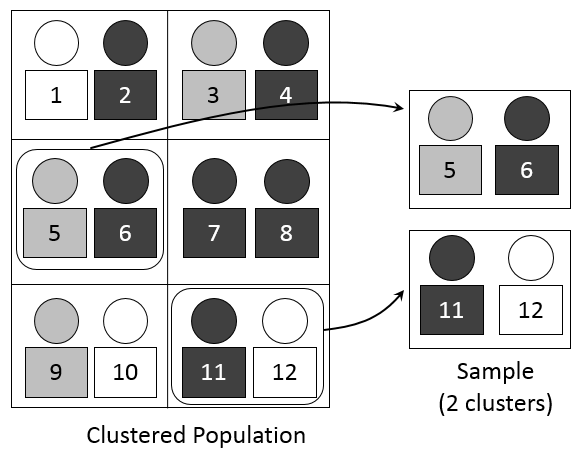
Let’s work through how we might use cluster sampling. While creating a list of all students in your state would be next to impossible, you could easily create a list of all school libraries in your state. Then, you could draw a random sample of elementary schools (your cluster) and then draw another random sample of elements (in this case, students) from each of the programs you randomly selected from the list of all programs.
Cluster sampling often works in stages. In this example, we sampled in two stages—(1) library programs and (2) elementary-age students at each program we selected. However, we could add another stage if it made sense to do so. We might randomly select (1) school districts in the province (2) schools by type (urban or rural), and (3) individual elementary-age students. As you might have guessed, sampling in multiple stages does introduce a greater possibility of error. Each stage is subject to its own sampling problems. But, cluster sampling is nevertheless a highly efficient method.
Jessica Holt and Wayne Gillespie (2008)[4] used cluster sampling in their study of students’ experiences with violence in intimate relationships. Specifically, the researchers randomly selected 14 classes on their campus and then drew a random sub-sample of students from those classes. But you probably know from your experience with college classes that not all classes are the same size. So, if Holt and Gillespie had simply randomly selected 14 classes and then selected the same number of students from each class to complete their survey, then students in the smaller of those classes would have had a greater chance of being selected for the study than students in the larger classes. Keep in mind, with random sampling the goal is to make sure that each element has the same chance of being selected. When clusters are of different sizes, as in the example of sampling college classes, researchers often use a method called probability proportionate to size (PPS). This means that they take into account that their clusters are of different sizes. They do this by giving clusters different chances of being selected based on their size so that each element within those clusters winds up having an equal chance of being selected.
To summarize, probability samples allow a researcher to make conclusions about larger groups. Probability samples require a sampling frame from which elements, usually human beings, can be selected at random from a list. The use of random selection reduces the error and bias present in non-probability samples, which we will discuss in greater detail in section 10.3, though some error will always remain. In relying on a random number table or generator, researchers can state that their sample is more likely to represent the population from which it was drawn. This strength is common to all probability sampling approaches summarized in Table 10.2.
Sample type | Description |
Simple random | Researcher randomly selects elements from sampling frame. |
Systematic | Researcher selects every kth element from sampling frame. |
Stratified | Researcher creates subgroups then randomly selects elements from each subgroup. |
Cluster | Researcher randomly selects clusters then randomly selects elements from selected clusters. |
In determining which probability sampling approach makes the most sense for your project, it helps to know more about your population. A simple random sample and systematic sample are relatively similar to carry out. They both require a list all elements in your sampling frame. Systematic sampling is slightly easier in that it does not require you to use a random number generator, instead using a sampling interval that is easy to calculate by hand.
However, the relative simplicity of both approaches is counterweighted by their lack of sensitivity to characteristics of your population. Stratified samples can better account for periodicity by creating strata that reduce or eliminate its effects. Stratified sampling also ensure that smaller subgroups are included in your sample, thereby making your sample more representative of the overall population. While these benefits are important, creating strata for this purpose requires having information about your population before beginning the sampling process. In our student example, we would need to know which students are full-time or part-time, graduate or undergraduate, in order to make sure our sample contained the same proportions. Would you know if someone was a graduate student or part-time student, just based on their email address? If the true population parameters are unknown, stratified sampling becomes significantly more challenging.
Common to each of the previous probability sampling approaches is the necessity of using a real list of all elements in your sampling frame. Cluster sampling is different. It allows a researcher to perform probability sampling in cases for which a list of elements is not available or feasible to create. Cluster sampling is also useful for making claims about a larger population (in our previous example, all elementary-age students within a province). However, because sampling occurs at multiple stages in the process, (in our previous example, at the district and student level), sampling error increases. For many researchers, the benefits of cluster sampling outweigh this weaknesses.
Matching recruitment and sampling approach
Recruitment must match the sampling approach you choose in section 10.2. For many students, that will mean using recruitment techniques most relevant to availability sampling. These may include public postings such as flyers, mass emails, or social media posts. However, these methods would not make sense for a study using probability sampling. Probability sampling requires a list of names or other identifying information so you can use a random process to generate a list of people to recruit into your sample. Posting a flyer or social media message means you don’t know who is looking at the flyer, and thus, your sample cannot be considered randomly drawn. Probability sampling often requires knowing how to contact specific participants. For example, you may contact potential participants via phone and email. Even then, it’s important to note that not everyone you contact will enter your study. We will discuss more about evaluating the quality of your sample in section 10.3.
Key Takeaways
- Probability sampling approaches are more accurate when the researcher wants to generalize from a smaller sample to a larger population. However, non-probability sampling approaches are often more feasible. You will have to weigh advantages and disadvantages of each when designing your project.
- There are many kinds of probability sampling approaches, though each require you know some information about people who potentially would participate in your study.
- Probability sampling also requires that you assign people within the sampling frame a number and select using a truly random process.
- Most often, action researchers and student researchers use non-probability convenience sampling because other methods are simply not feasible within the scope of their projects.
Exercises
Building on the step-by-step sampling plan from the exercises in section 10.1:
- Identify one of the sampling approaches listed in this chapter that might be appropriate to answering your question and list the strengths and limitations of it.
- Describe how you will recruit your participants and how your plan makes sense with the sampling approach you identified.
Examine one of the empirical articles from your literature review.
10.3 Sample quality
Learning Objectives
Learners will be able to…
- Assess whether your sampling plan is likely to produce a sample that is representative of the population you want to draw conclusions about
- Identify the considerations that go into producing a representative sample and determining sample size
- Distinguish between error and bias in a sample and explain the factors that can lead to each
Okay, so you’ve chosen where you’re going to get your data (setting), what characteristics you want and don’t want in your sample (inclusion/exclusion criteria), and how you will select and recruit participants (sampling approach and recruitment). That means you are done, right? (I mean, there’s an entire section here, so probably not.) Even if you make good choices and do everything the way you’re supposed to, you can still draw a poor sample. If you are investigating a research question using quantitative methods, the best choice is some kind of probability sampling, but aside from that, how do you know a good sample from a bad sample? As an example, we’ll use a bad sample the original author collected as part of a research project that didn’t go so well. Hopefully, your sampling will go much better than his did, but we can always learn from what didn’t work.
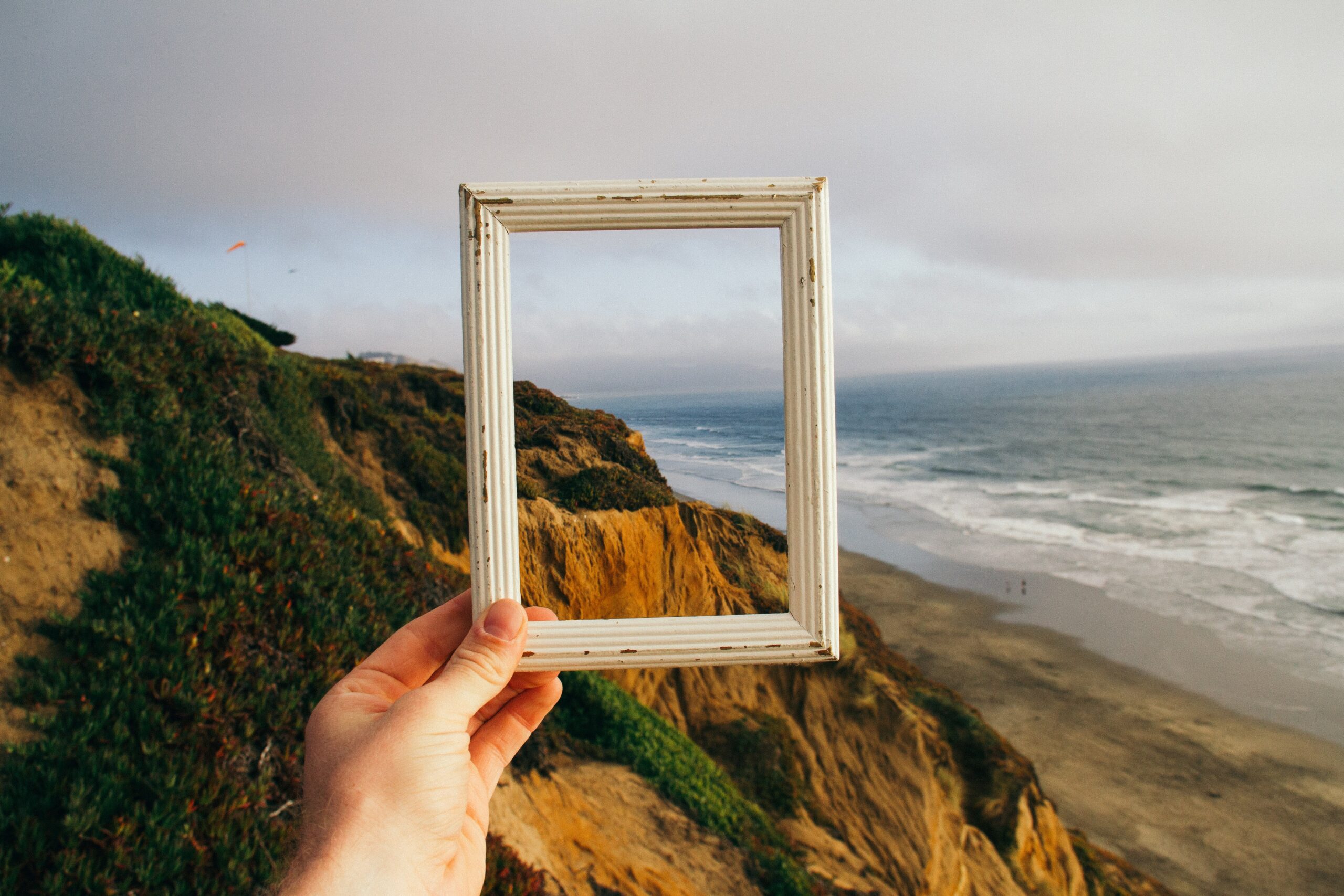
Representativeness
A representative sample is, “a sample that looks like the population from which it was selected in all respects that are potentially relevant to the study” (Engel & Schutt, 2011).[5] For his study on how much it costs to get an LCSW in each state, he did not get a sample that looked like the overall population to which he wanted to generalize. His sample had a few states with more than ten responses and most states with no responses. That does not look like the true distribution of social workers across the country. He could compare the number of social workers in each state, based on data from the National Association of Social Workers, or the number of recent clinical MSW graduates from the Council on Social Work Education. More than that, he could see whether his sample matched the overall population of clinical social workers in gender, race, age, or any other important characteristics. Sadly, it wasn’t even close. So, he wasn’t able to use the data to publish a report that would generalize to all LCSW.
That said, we still had potentially interesting data to analyze. While that data may only be relevant to the respondents on this particular project, it might still inform other researchers as they think about sampling, survey construction, and data analysis. This highlight a broader problem in research publishing, and that is the bias of journal editors and reviewers toward representativeness and significance in quantitative research. Because of that bias, we lose access to a wealth of information that might help us design better studies.
Exercises
Critique the representativeness of the sample you are planning to gather.
- Will the sample of people (or documents) look like the population to which you want to generalize?
- Specifically, what characteristics are important in determining whether a sample is representative of the population? How do these characteristics relate to your research question?
- Thinking back to your research goal, is representativeness and generalizability even necessary for the work you want to do?
Consider returning to this question once you have completed the sampling process and evaluate whether the sample in your study was similar to what you designed in this section.
Many of my students erroneously assume that using a probability sampling technique will guarantee a representative sample. This is not true. Engel and Schutt (2011) identify that probability sampling increases the chance of representativeness; however, it does not guarantee that the sample will be representative. If a representative sample is important to your study, it would be best to use a sampling approach that allows you to control the proportion of specific characteristics in your sample. For instance, stratified random sampling allows you to control the distribution of specific variables of interest within your sample. However, that requires knowing information about your participants before you hand them surveys or expose them to an experiment.
In the previous study, if the author wanted to make sure he had a certain number of people from each state (state being the strata), making the proportion of social workers from each state in his sample similar to the overall population, he would need to know which email addresses were from which states. That was not information he had. So, instead he conducted simple random sampling and randomly selected 5,000 of 100,000 email addresses on the NASW list. There was less of a guarantee of representativeness, but whatever variation existed between his sample and the population would be due to random chance. This would not be true for an availability or convenience sample. While these sampling approaches are common for student projects, they come with significant limitations in that variation between the sample and population may be due to factors other than chance. We will discuss these non-random differences later in the chapter when we talk about bias. For now, just remember that the representativeness of a sample is helped by using random sampling, though it is not a guarantee.
Exercises
- Before you start sampling, do you know enough about your sampling frame to use stratified random sampling, which increases the potential of getting a representative sample?
- Do you have enough information about your sampling frame to use another probability sampling approach like simple random sampling or cluster sampling?
- If little information is available on which to select people, are you using availability sampling? Remember that availability sampling is okay if it is the only approach that is feasible for the researcher, but it comes with significant limitations when drawing conclusions about a larger population.
Assessing representativeness should start prior to data collection. The previous author mentioned that he drew his sample from the NASW email list, which (like most organizations) they sell to advertisers when companies or researchers need to reach social workers. How representative of his population is that sampling frame? Well, the first question to ask is what proportion of his sampling frame would actually meet his exclusion and inclusion criteria. Since his study focused specifically on clinical social workers, his sampling frame likely included social workers who were not clinical social workers, like macro social workers or social work managers. However, he knew, based on the information from NASW marketers, that many people who received his recruitment email would be clinical social workers or those working towards licensure, so he was satisfied with that. Anyone who didn’t meet his inclusion criteria and opened the survey would be greeted with clear instructions that this survey did not apply to them.
At the same time, he should have assessed whether the demographics of the NASW email list and the demographics of clinical social workers more broadly were similar. Unfortunately, this was not information he could gather. He had to trust that this was likely to going to be the best sample he could draw and the most representative of all social workers.
A key point about online surveys–while he was concerned about generalizability, even if we got thousands of responses there would always be the caveat that he didn’t know who filled out the survey. E-mails could have been misdirected, a spouse or student might have read the message and filled out the survey, or an online spider may have found the page and flooded the system with false data. At the end of the day, there will always be error in our data collection, and there will be in your research. Embrace that, admit it, and be prepared to deal with it as best you can.
Exercises
- Before you start, what do you know about your setting and potential participants?
- Are there likely to be enough people in the setting of your study who meet the inclusion criteria?
You want to avoid throwing out half of the surveys you get back because the respondents aren’t a part of your target population. This is a common error I see in student proposals.
Many of you will sample people from your school or district, like students, teachers, or staff. Let’s say you work for a school district, and you wanted to study children who have experienced abuse. Walking through the steps here might proceed like this:
- Think about or ask your coworkers how many of the students have experienced this issue. If it’s common, then students in your school would probably make a good sampling frame for your study. If not, then you may want to adjust your research question or consider a different sample. You could also change your target population to be more representative with your sample. For example, while your school’s students may not be representative of all children who have survived abuse, they may be more representative of abuse survivors in your province, region, or city. In this way, you can draw conclusions about a smaller population, rather than everyone in the world who is a victim of child abuse.
- Think about those characteristics that are important for individuals in your sample to have or not have. Obviously, the variables in your research question are important, but so are the variables related to it. Take a look at the empirical literature on your topic. Are there different demographic characteristics or covariates that are relevant to your topic?
- All of this assumes that you can actually access information about your sampling frame prior to collecting data. This is a challenge in the real world. Even if you ask around your school about student characteristics, there is no way for you to know for sure until you complete your study whether it was the most representative sampling frame you could find. When in doubt, go with whatever is feasible and address any shortcomings in sampling within the limitations section of your research report. A good project is a done project.
- While using a probability sampling approach helps with sample representativeness, it does not guarantee it. Due to random variation, samples may differ across important characteristics. If you can feasibly use a probability sampling approach, particularly stratified random sampling, it will help make your sample more representative of the population.
- Even if you choose a sampling frame that is representative of your population and use a probability sampling approach, there is no guarantee that the sample you are able to collect will be representative. Sometimes, people don’t respond to your recruitment efforts. Other times, random chance will mean people differ on important characteristics from your target population. ¯\_(ツ)_/¯
In school samples, the small size of the pool of potential participants makes it very likely that your sample will not be representative of a broader target population. Sometimes, researchers look for specific outcomes connected with sub-populations for that reason. Not all school-based research is concerned with representativeness, and it is still worthwhile to pursue research that is relevant to only one location as its purpose is often to improve teaching practice.
Sample size
Let’s assume you have found a representative sampling frame, and that you are using one of the probability sampling approaches we reviewed in section 10.2. That should help you recruit a representative sample, but how many people do you need to recruit into your sample? As with many questions about sample quality, students should keep feasibility in mind. The easiest answer I’ve given as a professor is, “as many as you can, without hurting yourself.” While your quantitative research question would likely benefit from hundreds or thousands of respondents, that is not likely to be feasible for a student who is working full-time and in school full-time. Don’t feel like your study has to be perfect, but make sure you note any limitations in your final report. For example something like, ‘While I used non-probability sampling to create my participant group, I could have used probability sampling, including stratified random sampling, if I had the time and resources to do so. Unfortunately, I did nor.’
To the extent possible, you should gather as many people as you can in your sample who meet your criteria. But why? Let’s think about an example you probably know well. Have you ever watched the TV show Family Feud? Each question the host reads off starts with, “we asked 100 people…” Believe it or not, Family Feud uses simple random sampling to conduct their surveys of the American public. Part of the challenge on Family Feud is that people can usually guess the most popular answers, but those answers that only a few people chose are much harder. They seem bizarre, and are more difficult to guess. That’s because 100 people is not a lot of people to sample. Essentially, Family Feud is trying to measure what the answer is for all 327 million people in the United States by asking 100 of them. As a result, the weird and idiosyncratic responses of a few people are likely to remain on the board as answers, and contestants have to guess answers fewer and fewer people in the sample provided. In a larger sample, the oddball answers would likely fade away and only the most popular answers would be represented on the game show’s board.
In the previous author’s ill-fated study of clinical social workers, he received 87 complete responses. That is far below the hundred thousand licensed or license-eligible clinical social workers. Moreover, since he wanted to conduct state-by-state estimates, there was no way he had enough people in each state to do so. For student projects, samples of 50-100 participants are more than enough to write a paper (or start a game show), but for projects in the real world with real-world consequences, it is important to recruit the appropriate number of participants. For example, if your agency conducts a community scan of people in your service area on what services they need, the results will inform the direction of your agency, which grants they apply for, who they hire, and its mission for the next several years. Being overly confident in your sample could result in wasted resources for clients.
So what is the right number? Theoretically, we could gradually increase the sample size so that the sample approaches closer and closer to the total size of the population (Bhattacherjeee, 2012).[6] But as we’ve talked about, it is not feasible to sample everyone. How do we find that middle ground? To answer this, we need to understand the sampling distribution. Imagine in your survey of the local school community, you took three different probability samples, and for each sample, you measured whether people attended a social event at the school. If each random sample was truly representative of the population, then your rate of attendance from the three random samples would be about the same and equal to the true value in the population.
But this is extremely unlikely, given that each random sample will likely constitute a different subset of the population, and hence, the rate of attendance you measured may be slightly different from sample to sample. Think about the sample you collect as existing on a distribution of infinite possible samples. Most samples you collect will be close to the population mean but many will not be. The degree to which they differ is associated with how much the subject you are sampling about varies in the population. In our example, samples will vary based on how varied the incidence of social engagement in schools is from person to person. The difference between the rate we find and the rate for our overall population is called the sampling error.
An easy way to minimize sampling error is to increase the number of participants in your sample, but in actuality, minimizing sampling error relies on a number of factors outside of the scope of a basic student project. You can see this online textbook for more examples on sampling distributions or take an advanced methods course at your university, particularly if you are considering becoming a quantitative researcher. Increasing the number of people in your sample also increases your study’s power, or the odds you will detect a significant relationship between variables when one is truly present in your sample. If you intend on publishing the findings of your student project, it is worth using a power analysis to determine the appropriate sample size for your project. You can follow this excellent video series from the Center for Open Science on how to conduct power analyses using free statistics software. You may be surprised to find out that there is a point at which you adding more people to your sample will not make your study any better.
Honestly, the previous author did not do a power analysis for his study. Instead, he asked for 5,000 surveys with the hope that 1,000 would come back. Given that only 87 came back, a power analysis conducted after the survey was complete would likely to reveal that I did not have enough statistical power to fully answer his research questions. For your projects, try to get as many respondents as you feasibly can, but don’t worry too much about not reaching the optimal amount of people to maximize the power of your study unless you goal is to publish something that is generalizable to a large population.
A final consideration is which statistical test you plan to use to analyze your data. We have not covered statistics yet, though we will provide a brief introduction to basic statistics in this textbook. For now, remember that some statistical tests have a minimum number of people that must be present in the sample in order to conduct the analysis. You will complete a data analysis plan before you begin your project and start sampling, so you can always increase the number of participants you plan to recruit based on what you learn in the next few chapters.
Exercises
- How many people can you feasibly sample in the time you have to complete your project?
Bias
One of the interesting things about surveying professionals is that sometimes, they email you about what they perceive to be a problem with your study (I do this ALL the time!). The previous author got an email from a well-meaning participant in his LCSW study saying that his results were going to be biased! She pointed out that respondents who had been in practice a long time, before clinical supervision was required, would not have paid anything for supervision. This would lead him to draw conclusions that supervision was cheap, when in fact, it was expensive. His email back to her explained that she hit on one of his hypotheses, that social workers in practice for a longer period of time faced fewer costs to becoming licensed. Her email reinforced that he needed to account for the impact of length of practice on the costs of licensure he found across the sample. She was right to be on the lookout for bias in the sample. That said, as a researcher you should generally try NOT to frustrate your participants–while some may e-mail you, many others will just turn away.
One of the key questions you can ask is if there is something about your process that makes it more likely you will select a certain type of person for your sample, making it less representative of the overall population. In his project, it’s worth thinking more about who is more likely to respond to an email advertisement for a research study. He knew that his work email and personal email filter out advertisements, so it’s unlikely he would even have seen the recruitment for his own study (probably something he should have thought about before using grant funds to sample the NASW email list). Perhaps an older demographic that does not screen advertisements as closely, or those whose NASW account was linked to a personal email with fewer junk filters would be more likely to respond. To the extent he made conclusions about clinical social workers of all ages based on a sample that was biased towards older social workers, his results would be biased. This is called selection bias, or the degree to which people in his sample differ from the overall population.
Another potential source of bias here is nonresponse bias. Because people do not often respond to email advertisements (no matter how well-written they are), his sample is likely to be representative of people with characteristics that make them more likely to respond. They may have more time on their hands to take surveys and respond to their junk mail. To the extent that the sample is comprised of social workers with a lot of time on their hands (who are those people?) his sample would be biased and not representative of the overall population.
It’s important to note that both bias and error describe how samples differ from the overall population. Error describes random variations between samples, due to chance. Using a random process to recruit participants into a sample means you will have random variation between the sample and the population. Bias creates variance between the sample and population in a specific direction, such as towards those who have time to check their junk mail. Bias may be introduced by the sampling method used or due to conscious or unconscious bias introduced by the researcher (Rubin & Babbie, 2017).[7] A researcher might select people who “look like good research participants,” in the process transferring their unconscious biases to their sample. They might exclude people from the sampling from who “would not do well with the intervention.” Careful researchers can avoid these, but unconscious and structural biases can be challenging to root out.
Exercises
- Identify potential sources of bias in your sample and brainstorm ways you can minimize them, if possible.
Critical considerations
Think back to you undergraduate degree. Did you ever participate in a research project as part of an introductory psychology or sociology course? Social science researchers on college campuses have a luxury that researchers elsewhere may not share—they have access to a whole bunch of (presumably) willing and able human guinea pigs. But that luxury comes at a cost—sample representativeness. One study of top academic journals in psychology found that over two-thirds (68%) of participants in studies published by those journals were based on samples drawn in the United States (Arnett, 2008).[8] Further, the study found that two-thirds of the work that derived from US samples published in the Journal of Personality and Social Psychology was based on samples made up entirely of American undergraduate students taking psychology courses.
These findings certainly raise the question: What do we actually learn from social science studies and about whom do we learn it? That is exactly the concern raised by Joseph Henrich and colleagues (Henrich, Heine, & Norenzayan, 2010),[9] authors of the article “The Weirdest People in the World?” In their piece, Henrich and colleagues point out that behavioral scientists very commonly make sweeping claims about human nature based on samples drawn only from WEIRD (Western, Educated, Industrialized, Rich, and Democratic) societies, and often based on even narrower samples, as is the case with many studies relying on samples drawn from college classrooms. As it turns out, robust findings about the nature of human behavior and learning when it comes to fairness, cooperation, visual perception, trust, and other behaviors are based on studies that excluded participants from outside the United States and sometimes excluded anyone outside the college classroom (Begley, 2010).[10] This certainly raises questions about what we really know about human behavior as opposed to US resident or US undergraduate behavior. Of course, not all research findings are based on samples of WEIRD folks like college students. But even then, it would behoove us to pay attention to the population on which studies are based and the claims being made about those to whom the studies apply.
Another thing to keep in mind is that just because a sample may be representative in all respects that a researcher thinks are relevant, there may be relevant aspects that didn’t occur to the researcher when she was drawing her sample. You might not think that a person’s phone would have much to do with their voting preferences, for example. But had pollsters making predictions about the results of the 2008 presidential election not been careful to include both cell phone-only and landline households in their surveys, it is possible that their predictions would have underestimated Barack Obama’s lead over John McCain because Obama was much more popular among cell phone-only users than McCain (Keeter, Dimock, & Christian, 2008).[11] This is another example of bias.
Putting it all together
So how do we know how good our sample is or how good the samples gathered by other researchers are? While there might not be any magic or always-true rules we can apply, there are a couple of things we can keep in mind as we read the claims researchers make about their findings.
First, remember that sample quality is determined only by the sample actually obtained, not by the sampling method itself. A researcher may set out to administer a survey to a representative sample by correctly employing a random sampling approach with impeccable recruitment materials. But, if only a handful of the people sampled actually respond to the survey, the researcher should not make claims that their sampling went according to plan.
Another thing to keep in mind, as demonstrated by the preceding discussion, is that researchers may be drawn to talking about implications of their findings as though they apply to some group other than the population actually sampled. Whether the sampling frame does not match the population or the sample and population differ on important criteria, the resulting sampling error can lead to bad science.
We’ve talked previously about the perils of generalizing social science findings from graduate students in the United States and other Western countries to all cultures in the world, imposing a Western view as the right and correct view of the social world. As consumers of theory and research, it is our responsibility to be attentive to this sort of (likely unintentional) bait and switch. And as researchers, it is our responsibility to make sure that we only make conclusions from samples that are representative. A larger sample size and probability sampling can improve the representativeness and generalizability of the study’s findings to larger populations, though neither are guarantees.
Finally, keep in mind that a sample allowing for comparisons of theoretically important concepts or variables is certainly better than one that does not allow for such comparisons. In a study based on a nonrepresentative sample, for example, we can learn about the strength of our social theories by comparing relevant aspects of social processes. We talked about this as theory-testing in Chapter 8.
At their core, questions about sample quality should address who has been sampled, how they were sampled, and for what purpose they were sampled. Being able to answer those questions will help you better understand, and more responsibly interpret, research results. For your study, keep the following questions in mind.
- Are your sample size and your sampling approach appropriate for your research question?
- How much do you know about your sampling frame ahead of time? How will that impact the feasibility of different sampling approaches?
- What gatekeepers and stakeholders are necessary to engage in order to access your sampling frame?
- Are there any ethical issues that may make it difficult to sample those who have first-hand knowledge about your topic?
- Does your sampling frame look like your population along important characteristics? Once you get your data, ask the same question of the sample you successfully recruit.
- What about your population might make it more difficult or easier to sample?
- How many people can you feasibly sample in the time you have to complete your project?
- Are there steps in your sampling procedure that may bias your sample to render it not representative of the population?
- If you want to skip sampling altogether, are there sources of secondary data you can use? Or might you be able to answer you questions by sampling documents or media, rather than people?
Key Takeaways
- The sampling plan you implement should have a reasonable likelihood of producing a representative sample. Student projects are given more leeway with nonrepresentative samples, and this limitation should be discussed in the research report.
- If practical, researchers should conduct a power analysis to determine sample size, though quantitative student projects should endeavor to recruit as many participants as possible. Sample size impacts representativeness of the sample, its power, and which statistical tests can be conducted.
- The sample you collect is one of an infinite number of potential samples that could have been drawn. To the extent the data in your sample varies from the data in the entire population, it includes some error or bias. Error is the result of random variations. Bias is systematic error that pushes the data in a given direction.
- Even if you do everything right, there is no guarantee that you will draw a good sample. Flawed samples are okay to use as examples in the classroom, but the results of your research would have limited generalizability beyond your specific participants.
- Historically, samples were drawn from dominant groups and generalized to all people. This shortcoming is a limitation of some social science literature and should be considered a colonialist scientific practice.
- I clearly need a snack. ↵
- Johnson, P. S., & Johnson, M. W. (2014). Investigation of “bath salts” use patterns within an online sample of users in the United States. Journal of psychoactive drugs, 46(5), 369-378. ↵
- While i have left the original text here, as I think it serves as a good example of the comoplexities of research sampling, I do plan to update it down the line to tell the story on my own doctoral thesis. There are lots of similarities, however, I still found value in the work, which was well received by reviewers. ↵
- Holt, J. L., & Gillespie, W. (2008). Intergenerational transmission of violence, threatened egoism, and reciprocity: A test of multiple psychosocial factors affecting intimate partner violence. American Journal of Criminal Justice, 33, 252–266. ↵
- Engel, R. & Schutt (2011). The practice of research in social work (2nd ed.). California: SAGE ↵
- Bhattacherjee, A. (2012). Social science research: Principles, methods, and practices. Retrieved from: https://scholarcommons.usf.edu/cgi/viewcontent.cgi?article=1002&context=oa_textbooks ↵
- Rubin, C. & Babbie, S. (2017). Research methods for social work (9th edition). Boston, MA: Cengage. ↵
- Arnett, J. J. (2008). The neglected 95%: Why American psychology needs to become less American. American Psychologist, 63, 602–614. ↵
- Henrich, J., Heine, S. J., & Norenzayan, A. (2010). The weirdest people in the world? Behavioral and Brain Sciences, 33, 61–135. ↵
- Newsweek magazine published an interesting story about Henrich and his colleague’s study: Begley, S. (2010). What’s really human? The trouble with student guinea pigs. Retrieved from http://www.newsweek.com/2010/07/23/what-s-really-human.html ↵
- Keeter, S., Dimock, M., & Christian, L. (2008). Calling cell phones in ’08 pre-election polls. The Pew Research Center for the People and the Press. Retrieved from http://people-press.org/files/legacy-pdf/cell-phone-commentary.pdf ↵
entity that a researcher wants to say something about at the end of her study (individual, group, or organization)
the entities that a researcher actually observes, measures, or collects in the course of trying to learn something about her unit of analysis (individuals, groups, or organizations)
the larger group of people you want to be able to make conclusions about based on the conclusions you draw from the people in your sample
the list of people from which a researcher will draw her sample
the people or organizations who control access to the population you want to study
an administrative body established to protect the rights and welfare of human research subjects recruited to participate in research activities conducted under the auspices of the institution with which it is affiliated
Inclusion criteria are general requirements a person must possess to be a part of your sample.
characteristics that disqualify a person from being included in a sample
the process by which the researcher informs potential participants about the study and attempts to get them to participate
the group of people you successfully recruit from your sampling frame to participate in your study
sampling approaches for which a person’s likelihood of being selected from the sampling frame is known
sampling approaches for which a person’s likelihood of being selected for membership in the sample is unknown
researcher gathers data from whatever cases happen to be convenient or available
(as in generalization) to make claims about a large population based on a smaller sample of people or items
selecting elements from a list using randomly generated numbers
the units in your sampling frame, usually people or documents
selecting every kth element from your sampling frame
the distance between the elements you select for inclusion in your study
the tendency for a pattern to occur at regular intervals
dividing the study population into subgroups based on a characteristic (or strata) and then drawing a sample from each subgroup
the characteristic by which the sample is divided in stratified random sampling
a sampling approach that begins by sampling groups (or clusters) of population elements and then selects elements from within those groups
in cluster sampling, giving clusters different chances of being selected based on their size so that each element within those clusters has an equal chance of being selected
a sample that looks like the population from which it was selected in all respects that are potentially relevant to the study
the set of all possible samples you could possibly draw for your study
The difference between what you find in a sample and what actually exists in the population from which the sample was drawn.
the odds you will detect a significant relationship between variables when one is truly present in your sample
the degree to which people in my sample differs from the overall population
The bias that occurs when those who respond to your request to participate in a study are different from those who do not respond to you request to participate in a study.