16.4 Computer Models of the Earth System
There Are Models and Then There Are Models
Models are key components of many kinds of studies, not just studies of the Earth system. Unfortunately, there are many misconceptions about what we mean by “model,” and how we use models. Discussions can also become complicated in a hurry, because while the main ideas may be simple enough, as soon as you start to take all of the components into consideration, there’s a lot of detail to think about! Below are a series of examples to show you how we think about and use models.
Models, Version 1.0
You may have seen models of volcanoes before—papier mâché or clay cone shapes that erupt a foamy liquid when someone pours vinegar on the baking soda hidden in the cone, or pours cola on Mentos candies. If the model-maker is paying attention to detail, they might make the cone extra realistic, or find a way to colour the foamy liquid a lava shade of red. This type of model is fun to make and demonstrate (less fun to clean up), but not necessarily used to answer scientific questions.
Models, Version 2.0
Now imagine the process of filling a bathtub. You might not pay much attention to what you’re doing, but in one way or another you are measuring temperature (perhaps putting your hand under the running water), and gauging flow rate (e.g., telling how fast the water is flowing by looking at how wide the stream of water is coming from the tap). The entire time you’re managing the first two tasks, you are also factoring in a knowledge of how much water your tub can hold, and using that to adjust your flow rates so you get a comfortable water temperature without overflowing the tub.
If you had to give a robot instructions on how to fill your tub, and when it was done, you were obligated to jump in, you would want to carefully quantify (describe with numbers) things like what a comfortable temperature is, or how the robot should know how much to turn the taps. You’d have to tell the robot what the smallest reasonable volume of water is for a bath, not just the largest.
If you didn’t want to trust your own instructions entirely (what if someone is using water elsewhere in the house?), you might get the robot to measure the temperature and then tell it how to make adjustments in response to what it measures. (Remember to tell it to stir the water first.) If your robot was then programmed to take any set of initial conditions (starting conditions, such as a house guest having used a lot of your hot water), and turn that into a comfortable bath, you would have designed your own control system.
Models, Version 3.0
You have now developed an interest in examining the behavior of humans filling a bathtub. You program your robot to fill a bathtub the way you would normally do it yourself, but then add a twist. What if the person (robot) filling the tub had a nerve condition that made it hard for them to gauge temperature by touch? You make your robot do the process as a human would, but without measuring temperatures. The robot must use assumptions about how much to turn the taps, and have ways of gauging flow rates without touching the water. You try a range of initial conditions (e.g., a house guest has just flushed the toilet) and see how often experiments end with the robot drawing a bath with a safe and comfortable temperature.
Models, Version 4.0
At some point you might decide to skip the robot and physical bathtub altogether. Instead, you just tell a computer how big your tub is, the specifics of how your household plumbing system works, and how the flow rate changes when you turn the taps. You can then give the computer a set of scenarios (ensemble) to run one after the other (e.g., house guest flushing the toilet, dishwasher on, washing machine on, etc.), and go for lunch.
If you were to take this approach, you would need to be confident that you could program a very good description of your plumbing system, and confident of what happens when turning the taps. You would probably run tests to quantify those things (such as by turning the taps open by different amounts and measuring flow rate), and look at how others have managed the same problem. To be extra sure, you might try filling the tub yourself under some of those scenarios, and verify that your model (because that’s what you’ve built here) gives results that match real life.
If you come to conclusions that you want to share with others, you will not only show the results of your model experiments, but also explain how you made sure your model was designed to give results that are meaningful (tied to reality). You would probably be asked how your model applies to other households, given that you designed it with your own plumbing system in mind. You might go back and try the whole set of experiments over again for different kinds of plumbing systems. If you find that it doesn’t make a big difference to the main outcomes of the experiment, you could tell people that your results were not sensitive to the type of plumbing system.
Earth-System Models
Earth-system interactions are so complex that it may be next to impossible to follow all of the connections and implications without help. So, scientists use Earth-system computer models to assist. Earth-system models incorporate knowledge of the many components of the Earth system in a way that makes it possible to test how important any one change is.
Earth-system models vary in how many aspects of the Earth system they include, and how detailed their representations of those aspects are. Models are designed to answer particular kinds of questions so their performance can be optimized; a study that is concerned only with large-scale global changes might not require a model with a highly detailed representation of Earth’s coastlines. It takes more time to run a complicated model, so this saves on computing resources.
When computer models are discussed, we acknowledge that there is a difference between measurements of the real world, and the output from the model. Modelers are careful to refer to measurements of the real word as data, and output from the model as results. (In the bathtub example, your measurements of how flow rate changes when you turn the taps would be data. The number of times the robot successfully draws a safe bath would be results.)
Distinguishing between data and results also helps to avoid confusion when using comparisons of model output to real-world measurements to gauge how realistic that model output is. (This is the difference between collecting data about how a human fills a tub under certain conditions, and comparing that to what the model does (results) under those same conditions.
What Are Computer Models, Exactly?
Computer models describe natural phenomena using mathematical equations. On the most basic level, computer models take some quantity—whether heat, water, or the concentration of a pollutant—and calculate how it moves through a system. The system is usually broken down into discrete cells for calculation purposes. Think about an image made of pixels. The model may calculate how materials move into one pixel and on to the next.
The “pixels” can represent actual space, but sometimes the model only looks at how a quantity changes through time. A computer model of the water volume in our bathtub could be limited to looking at how rapidly water flows in through the tap, and how rapidly it flows out through the drain. The entire bathtub is represented by a single “pixel.” This is fine if all we care about is how much water there is in total, not the water volume at different locations in the tub.
A study of wind-driven currents in a lake (which we will consider a very large bathtub for purposes of extending the analogy) must include information about the shape and depth of the lake to capture how friction at the lake bottom and along the sides affects water flow (Figure 16.28). Data about the lake shape and depth (Figure 16.28, top) is translated to a model grid (Figure 16.28, bottom). Calculations are done to see how wind and friction control how water moves into and out of each cell in the grid.
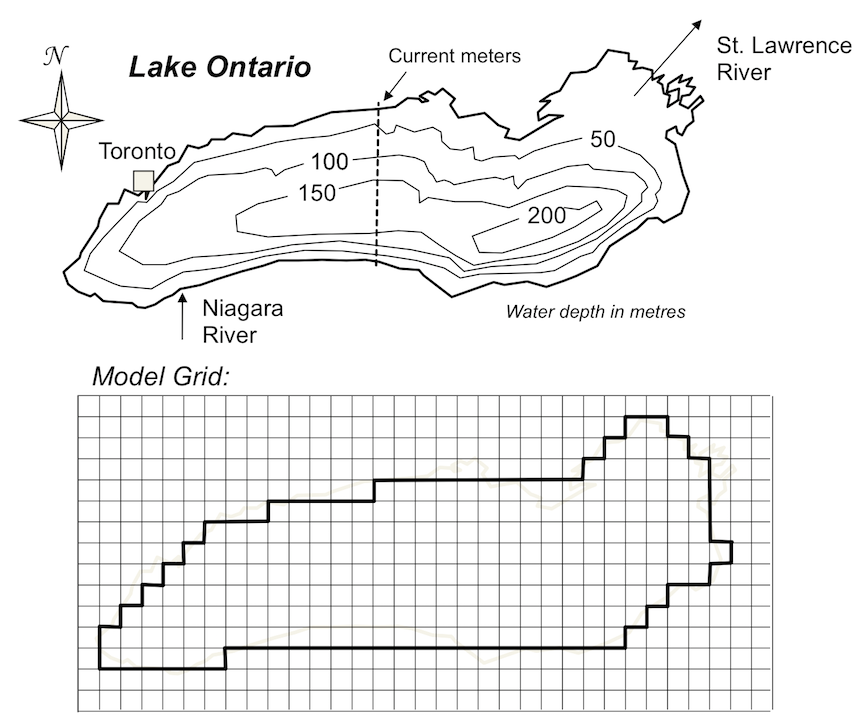
The model produces information about wave height (Figure 16.29, left) and shows the direction and speed of water flow across the lake using arrows of different sizes (Figure 16.29, right). If we want to know how a pollutant would move around the lake, we would specify where the pollutant is entering, and how much is added. It flows with the water, so by knowing how the water moves, we can predict where the pollutant would go, and at what concentrations.
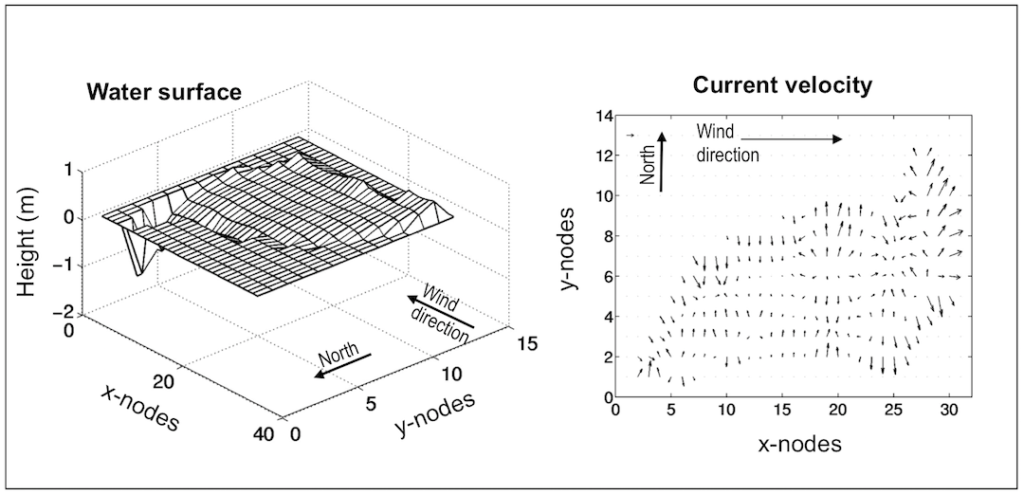
If the model is to be used to track the movement of a pollutant through the lake, it’s important to know that the model has done a good job of calculating how fast currents move (the current velocity). In this case, data from current meters in the lake can be compared to the current velocities that the model calculates (Figure 16.30). At first glance, the results and data might appear very different, but we have to be thoughtful about how we compare. The model does in fact capture the fact water flows northward near the margins of the lake, and southward in the middle. The current velocities are not exactly the same, however. This means that the model might do a reasonable job of predicting where the pollution went, but not as good a job at predicting how fast it got there.
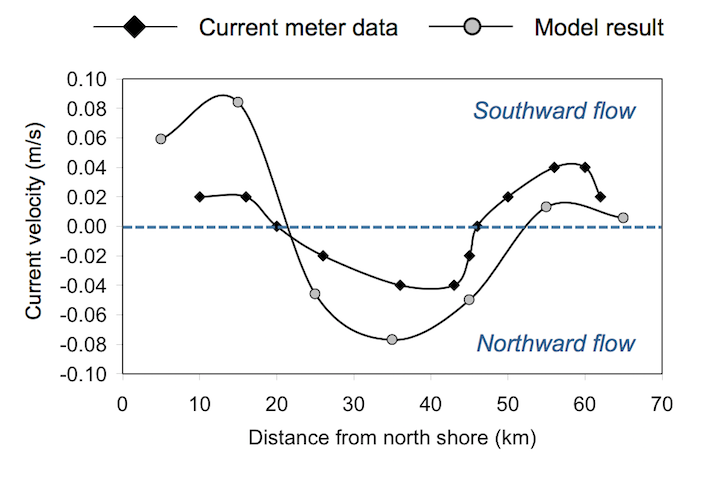
The model in this case had a relatively course representation of the lake geography (big pixels), so a first step would be to make the grid cells smaller to do a better job of simulating the shape of the lake, then look at the flow in finer detail. Another step would be to represent the lake water using a vertical stack of several grid cells to better capture the extent to which bottom friction and wind force affect the lake water at different depths, and to do a better job of representing the water depth.
An Example of Using a Computer Model to Study Past Earth-System Change
The Paleocene-Eocene Thermal Maximum (PETM) was a sudden global warming event that happened approximately 56 million years ago. There was interest in studying this event because its suddenness was thought to be a good analogy for the rapid changes happening in the Earth system today. Cores from ocean-floor rocks show that the oceans became so acidified during the PETM that calcium carbonate sediments dissolved over vast areas of the ocean floor, vanishing entirely from some regions. The same cores also showed a shift in the carbon-isotope composition of calcium carbonate sediments.
Both the acidification and the carbon-isotope shift indicated that a large amount of carbon was added to the Earth system to trigger the PETM. The problem was that there were a number of possible sources for the carbon, and thus a number of possible triggers for the event. Although scientists provided reasoned arguments for their favourite hypotheses (why their hypothesis was feasible), there was no way to know for sure which was the best answer.
To solve this problem, an Earth-system model was used that could test which scenario could best account for the pattern of dissolving calcium carbonate. It took into account the shape of ocean basins, ocean current circulation, and carbonate system chemistry in ocean water and in sediments. It also took into account changes in sediments once they were deposited.
The steps to using this model were the following:
1. Collect Data
A search was done to locate as many studies of ocean floor sampling sites as possible that had information about changes in the amount of calcium carbonate during the PETM. It was important to know not only how much calcium carbonate there was, but how deep the ocean was at the time those sediments were deposited.
2. Initial Conditions and Verification
The model was set up so that it did a good job of reproducing the distribution of calcium carbonate before the PETM happened. This was important to ensure that model scenarios began with a realistic set of conditions, and that the model itself was giving reasonable output.
3. Experiments
Each of the possible scenarios involved carbon coming from different sources in the Earth system. That meant each scenario could be represented in the model by adding to the atmosphere different amounts of carbon with different carbon isotope compositions. The more carbon a scenario required, the more the calcium carbonate sediments would have dissolved in real life.
The model was run for each different amount of carbon. For each scenario, the pattern of calcium carbonate sediments that the model gave was compared to the actual distribution of calcium carbonate sediments known from the data collected in Step 1.
4. Results and Analysis
In the end, the model showed that some of the scenarios did not even come close to matching the observations, either dissolving way too much calcium carbonate, or far too little. The model showed that two scenarios did come close to reproducing the pattern of calcium carbonate, and that one did a better job of matching the observations than the other. When it came time to write a report about the experiments, the scientists learned that newly published measurements from another study supported the scenario that the model suggested was best. It would have been acceptable to write a paper describing the model results, and which scenario worked best. However, also being able to comment about new supporting data meant there was a better chance of convincing other scientists that the model results were meaningful.
Predicting the Future of the Earth System with Models
Using models to investigate the Earth system requires careful consideration of how to build the model and run experiments. But it also requires skillful use of real-life measurements to set up the model, and to interpret and evaluate its results. The PETM model study was an example of how a model can be used to test hypotheses about past behaviour of the Earth system. There were data from before, during, and after the event to help set up the model and gauge its effectiveness.
Using Earth-system models to predict the future is a different kind of modeling challenge, because we don’t already know what the right answer is. The situation being modeled hasn’t happened yet. Scientists who try to predict the future of the Earth system have to think about things a bit differently in order to have some confidence in the reliability of their model outcomes:
1. Which forcing scenarios should be used?
A model used to predict the future of Earth’s climate will need input about what atmospheric greenhouse gas levels will be. That will depend on what actions humans take. Scientists deal with this unknown variable by testing multiple scenarios for greenhouse gas levels, such as what would happen if fossils fuels continue to be used as they have been, or alternatively, what would happen if we completely stopped using fossil fuels tomorrow. The scenarios they choose span a range of possibilities, including extreme cases, to make sure they understand what the possible range of outcomes could be.
2. Using present-day data for set-up and verification
Scientists use some of the data they have to set the model up so it’s a realistic representation of the Earth system at a particular time in history. They then test the model to see if it can reproduce a different set of data later in history. This is a way to see if the model can get the right answer for a time when we know the right answer. Finally, after determining that the model gives reasonable results for times when we know the right answer, it is run for future scenarios.
3. How do we know the results are meaningful?
To be confident about predictions of future Earth-system change, scientists may collaborate to run their scenarios on a variety of Earth-system models. Research groups each have their own ideas about how exactly the models should be set up. They may need to set it up a certain way to answer questions they’re interested in.
Models may be set up with more or less complicated mathematical representations of processes, or using different numbers of “pixels” to represent geographic details. The scientists who built the various models might disagree on what numbers to assign some of the variables, or even which parts of the Earth system are necessary to include. (Anyone who says scientists are colluding to get climate change models to give a certain result have never witnessed a room full of scientists attempting to agree on something.)
If, in spite of the wide range of ideas about how such models should work, the models produce similar results for a particular scenario, scientists can be more confident in those results.
4. How do we express confidence in the answers when we don’t actually know the right answer?
Scientists report uncertainty with their model results. As used in everyday language, uncertainty means we just don’t know something, or can’t say for sure. But for models, uncertainty is a number indicating the likelihood that a model result is within a certain range of values. It’s determined using methods that are themselves the product of entire research careers.
A meaningful discussion of uncertainty will concern a specific model or set of models, a specific variable, and include a specific range of values. It will also include information about how large the uncertainty is compared to the changes being investigated. (If your model predicts how tall a giraffe will be, and you’re confident in your answer to within a cm, that’s pretty good given how tall giraffes usually are. If these details are missing from the conversation, it’s a clue that “uncertainty” is being used in a common-language way rather than the way that modellers use it.
Reporting uncertainty is not exclusive to models predicting the future, but it’s particularly important for those models because of the great scrutiny Earth-system models receive when they are used to investigate future climate change.
References
Simons, T. J., & Schertzer, W. M. (1989) The circulation of Lake Ontario during the summer of 1982 and the winter of 1982/83. Burlington, ON: Environment Canada. Full text
Slingerland, R., & Kump, L. (2011). Mathematical Modeling of Earth’s Dynamical Systems: A Primer. Princeton, NJ: Princeton University Press.