7 Truncated studies: Was the trial stopped early for “overwhelming” evidence of benefit or futility?
Studies may be stopped early for efficacy as part of an ethical obligation to not expose participants to less effective treatment (or placebo) any longer than necessary. In other words, once it is sufficiently clear that an intervention is efficacious, there is reason to end the trial.
However stopping early runs the risk of overestimating the effect size of the intervention. The estimate of effect will randomly vary around the true effect over time (with more fluctuation with fewer events early in the trial), so interim looks may lead to premature stop due an exaggerated estimate of the true effect size.
Consider the following simulated trial where there is no true difference between the groups (i.e. RR = 1.0):
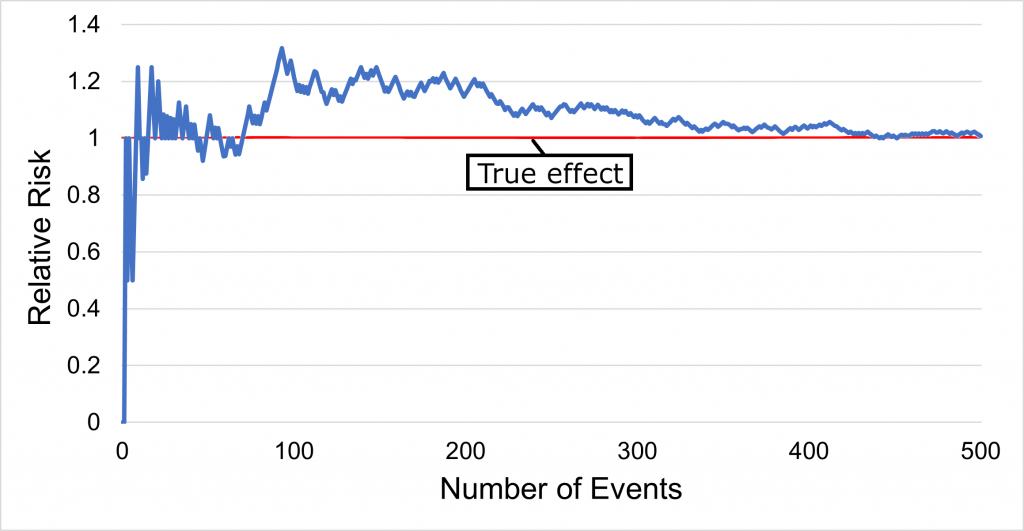
As depicted in Graph 2 above, there is random deviation from the true effect as events accumulate. If the trial had interim analyses for benefit every 100 events, and the threshold for statistical significance was kept at the standard p<0.05 without accounting for interim looks, then the trial may have stopped at 100 events when the RR was 1.3, which we know to be an exaggeration of the true effect (RR = 1.0, i.e. no effect).
As a simplified example, imagine studying a chess player and trying to assess if they are an above-average player (and by what margin) by judging their win percentage. One approach is to wait 50 matches, then assess their win percentage and judge accordingly. However, this could waste time as it might be unnecessary to wait that long if they are quite skilled (e.g. winning 90% of their first 10 games). So instead there could be an assessment of skill every 5 matches (up to a maximum of 50 matches). If they seem sufficiently impressive at one of these midpoint assessments, then the observation could be stopped. While this might save time, it also has a risk: if by pure chance the player goes on a win streak, then the observation is likely to end early. Even if our player is truly above-average in skill, an early stop is most likely to occur when they are on such a hot streak, consequently introducing bias into our assessment (e.g. assessing their win probability to be 80% due to the win streak, when in fact it is only 60%).
This is the major concern with stopping rules: there is a systematic tendency for an early stop to be an overestimation. While such precautions cannot prevent bias towards overestimation, they can help reduce the extent of this bias, as discussed below.
Checklist Questions
Was there a predefined interim analysis plan with a stopping rule? |
Did the stopping rule involve few interim looks and a stringent p-value (e.g. <0.001)? |
Did enough endpoint events occur? |
Was there a predefined interim analysis plan with a stopping rule?
Did the stopping rule involve few interim looks and a stringent p-value (e.g. <0.001)?
As the number of interim looks increases, then the probability of finding a false positive or overestimation also increases. This can be mitigated by (1) minimizing the number of interim looks and (2) having a stricter threshold for statistical significance that accounts for these multiple interim analyses.
Some common interim analysis strategies used (Schulz KF et al.) are:
Pocock: To keep the overall trial p-value threshold (alpha) = 0.05, the number of interim analyses are pre-defined & all have the same adjusted statistical significance threshold (i.e. p<0.029 for 2 planned analyses, p<0.016 for 5 planned analyses, and so forth).
Peto: Assign the final analysis p-value threshold = 0.05 (like in a conventional trial), but have a more stringent threshold (i.e. p<0.001) for the interim analyses.
O’Brien-Fleming: Begin with stringent interim analyses that start conservatively and then successively ease as they approach the final analysis (e.g. for 3 interim analyses & a final analysis, sequence of p-value thresholds 0.0001, 0.004, 0.019, 0.043)
Lan-DeMets: An adaptable approach where the significance level changes and analysis timing changes in accordance to previously observed information.
Did enough endpoint events occur?
Trials stopped early for benefit exaggerate the relative effect of an intervention by an average 29% compared with trials that conclude as planned (Bassler D et al.). As events accumulate, the fluctuations in effect size measures will become smaller and there will be less risk of bias (see graph above). Optimally ≥500 events (Bassler D et al.) should occur before stopping, after which the exaggeration decreases to an average of 12%.
For these reasons, skepticism is warranted for any relative risk reduction (RRR) ≥50% generated in truncated trials with <100 events (Pocock SJ et al., Montori VM et al.). The larger the number of events and the more plausible the RRR (e.g. ~20-30% is typical for the impact of cardiovascular pharmacotherapy on cardiovascular events), the more believable the results.
Relative risk (or risk ratio) is the risk in one group relative to (divided by) risk in another group. For example, if 10% in the treatment group and 20% in the placebo group have the outcome of interest, the relative risk in the treatment group is 0.5 (10% ÷ 20%; half) the risk in the placebo group. See here for a more detailed discussion.
Systematic deviation of an estimate from the truth (either an overestimation or underestimation) caused by a study design or conduct feature. See the Catalog of Bias for specific biases, explanations, and examples.
Randomized controlled trials are those in which participants are randomly allocated to two or more groups which are given different treatments.
A primary outcome is an outcome from which trial design choices are based (e.g. sample size calculations). Primary outcomes are not necessarily the most important outcomes.
Calculates the effect of an intervention via a fractional comparison with the comparator group (i.e. intervention group measure ÷ comparator group measure). Used for binary outcomes. Relative risk, odds ratio, or hazards ratio are all expressions of relative effect. For example, if the risk of developing neuropathy was 1% in the treatment group and 2% in the comparator group, then the relative risk is 0.5 (1 ÷ 2). See the Absolute Risk Differences and Relative Measures of Effect discussion here for more information.
The difference between two relative risks (RRs). If the intervention has a RR of 70% and the comparator a risk of 100%, then the relative risk reduction is 30% (100% - 70%).
This is the most accessible healthcare setting where generalist services are provided. For example, a family medicine clinic.
An outcome which consists of multiple component endpoints. For example, a cardiovascular composite may include stroke, myocardial infarction, and death.