84
Reproducibility and replicability are different but related concepts. There are important distinctions between the terms that need to be made when discussing how research workflows unfold across disciplines. Not all disciplines have historically paid attention reproducibility as it plays out within the scientific method but the increased use of computational tools and methods across all fields of research has made concepts around replicability and, in some cases, reproducibility more ubiquitously relevant.
Reproducibility
Reproducibility for research means the workflow and data used in the research project can be used to yield the same results. For research to be reproducible, a new team of researchers must be able to use the same experimental method, data, population, and general conditions of the first experiment to reproduce the same results. Improving reproducibility leads to increased rigour and quality of scientific outputs, and thus to greater trust in science. There has been a growing need and willingness to expose research workflows from initiation of a project and data collection right through to the interpretation and reporting of results.
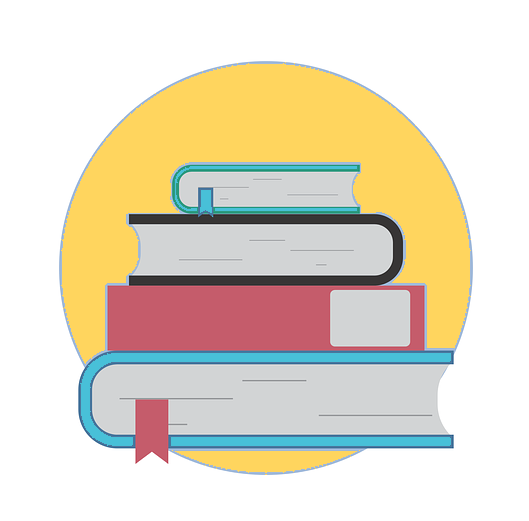
Dig Deeper
- Read a run down on reproducibility in science from The Nib.
Replicability
Replicability of research means that the same workflow is used by different people for a research project using a new dataset yielding different but expected results. This proves the transferability of the workflow. Replicability is more difficult to achieve as the results of a replicable research project are based on the reported methods of the original research using new data.
To get a better sense of the difference between these ideas, consider the image below. For something to be reproducible it needs to be possible for a group to repeat the same steps, with the same hypothesis, data and environment as before and get the same value. The value is the only unknown in this case. For something to be replicable a different group should be able to take the same context or population, research question, experimental design or approach, and analysis plan and make use of this approach to do new work with it on new data.

Reproducibility & Replication in the Humanities and Social Sciences
There is an ongoing debate around the degree of whether the concept of a “reproducibility” crisis can equally apply to the humanities and social science disciplines. While notions of one for one reproducibility do not always reflect the nature of work in these non-STEM disciplines, computational replicability is deeply relevant with the rise of digital scholarship. The ubiquitous use of digital tools and methods in modern research requires us to think about the ways in which our work, whether reliant on digital technologies or using digital technologies to augment existing workflows, will be possible to open, view, and manipulate in the future. A document written in proprietary software from twenty years ago may not be viewable on a modern device without intervention. Similarly a digital humanities project which uses a script to draw conclusions off of data will not be replicable unless that script is made available along with the data. This lack of persistence puts fundamental characteristics of humanities and social science research at risk and makes it difficult to build onto knowledge over time.

Incentives
Incentives to engage with open, reproducible, and replicable workflows vary greatly on both personal and professional levels. Disciplinary guidelines, tenure incentives, grant requirements, and peer workflows all contribute to varying degrees of planning and implementation of reproducible and replicable workflows. If your discipline has traditionally published monographs an open process of composition, feedback, and open resource lists might not be the norm and engaging with it may not be a high priority when the tenure process does not recognize it. Similarly a discipline in which results have traditionally been reported with descriptions of workflows, packaging an environment in which a workflow was run may seem like added labour that is not incorporated into existing timelines and workflows. Upskilling team members, particularly across interdisciplinary teams, can be an immense challenge.
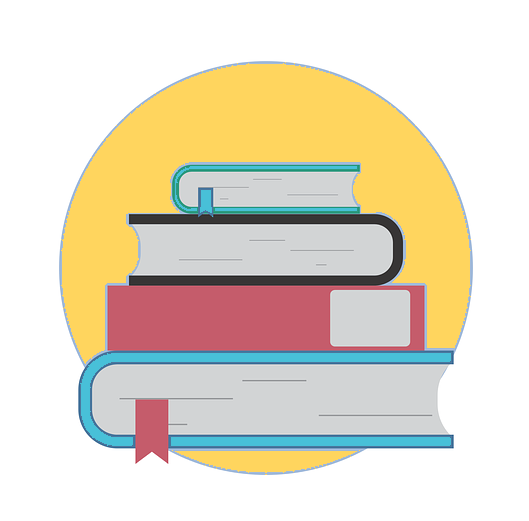
Dig Deeper
- Learn more about replicability in the humanities, by reading the following:
- Peels, R. (2019). Replicability and replication in the humanities. Research integrity and peer review, 4(1), 1-12. 10.1186/s41073-018-0060-4
- Peels, R., & Bouter, L. (2018). The possibility and desirability of replication in the humanities. Palgrave Communications, 4(1), 1-4. 10.1057/s41599-018-0149-x
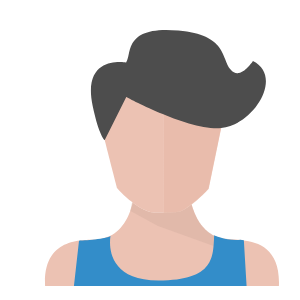
Scenario – Reproducibility
Let’s consider this scenario: you are starting a new collaborative research study and have engaged your partners in a conversation about how to make the results of the study reproducible. One of the project leads, an experienced researcher with many publications under their belt, made the following comments:
“All of the results will be in the paper, won’t people be able to reproduce our results from there? If they have any more questions they can reach out directly.”
How would you respond to them?
You may wish to note that having an extremely detailed description of the methods and workflows employed to reach the final result will not be sufficient in most cases to reproduce it. This can be due to several aspects, including different computational environments, differences in the software versions, implicit biases that were not clearly stated, etc. Additionally, spending the time and effort to put this together will increase both the scientific validity of the final results as well as minimize the time of re-running or extending it in further studies.